Generative AI (GenAI) startups have surged in recent years, spanning AI-driven content creation, coding assistants, conversational bots, and infrastructure APIs. But while their technological breakthroughs attract headlines, their monetization strategies pose a quieter but equally crucial challenge: how to price in a way that scales with value and usage.
In this exploration, we examine the pricing strategies of 28 leading GenAI firms across four categories: enterprise SaaS, developer APIs, consumer AI tools, and AI infrastructure. For each company, we unpack what they do, who they serve, how they price, and, critically, why they chose that approach. We look at the implications for customer value, revenue growth, and operational efficiency.
Why Pricing in GenAI Deserves a Closer Look
Unlike traditional SaaS, GenAI products often deliver value dynamically, based on usage, generated outputs, or even customer outcomes. Yet, many GenAI firms continue to default to SaaS-era norms. According to a recent analysis of 40 top AI startups:
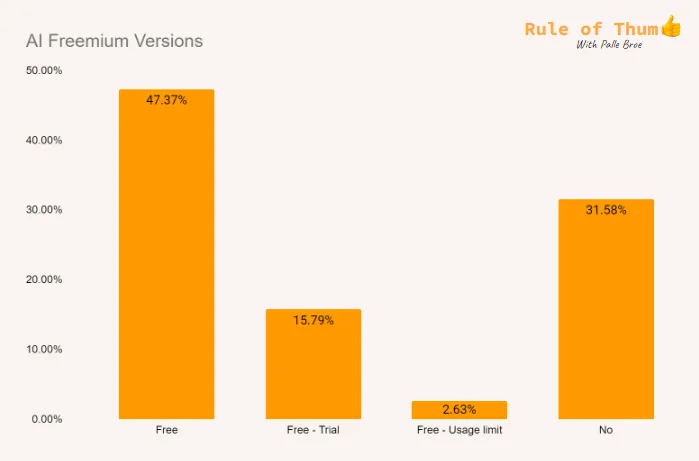
- Roughly 70% still use traditional subscription pricing, often per user.
- Pure usage-based pricing models are rare, despite GenAI’s inherently usage-driven value delivery.
- Hybrid models, blending seat-based licenses with usage-based fees, are emerging, offering a middle ground.
- A small but growing minority are experimenting with outcome-based pricing, where customers pay based on measurable success.
- About half offer a free plan or trial, either to reduce adoption friction or to accelerate data collection and model feedback.
These trends reflect a two-speed evolution in the market. The first wave of GenAI firms has largely adopted familiar SaaS conventions, simple to implement, familiar to buyers, and easy to forecast. But a second wave is now pushing boundaries, testing models that better align pricing with the AI-specific nature of usage, scale, and value realization.
Setting the Stage for the Profiles
Before diving into individual companies, it’s helpful to understand the broader shift in pricing logic underway:
- Subscription pricing (typically per-seat) remains dominant, especially among earlier-stage or enterprise-oriented startups.
- Hybrid pricing models, combining fixed licenses with metered usage, are gaining traction, especially in API-first or infrastructure-heavy offerings.
- Pure usage-based and outcome-based models remain niche, but their uptake is growing as firms look to reduce adoption barriers and better match value with cost.
This shift reflects more than just pricing mechanics. It’s a sign of deeper strategic choices: how risk is shared between vendor and customer, how value is communicated, and how scalable the revenue model is as usage grows.
What to Expect from the Company Profiles
In the sections that follow, we’ll break down the pricing strategies of 28 GenAI companies. Grouped by category, each profile examines:
- The company’s product and target market
- Its go-to-market motion and monetization model
- The rationale behind its pricing structure, whether for predictability, scalability, differentiation, or alignment with perceived value
You’ll see a range of approaches, from seat-based SaaS products that prioritize simplicity and sales efficiency, to usage-metered APIs that scale with demand, to novel success-fee models that tie cost directly to outcomes.
Each example provides a window into how today’s GenAI startups are balancing business model clarity with market experimentation.
What Comes Next
After the profiles, we’ll surface the common pricing patterns, strategic trade-offs, and monetization lessons that stand out across these 28 firms. Finally, we’ll map these insights to the 5-step pricing framework from Monetizely’s Price to Scale Vol2 Book, offering founders a structured path to refine or evolve their pricing strategy in this rapidly shifting landscape.
1. Jasper: AI Content for Marketing Teams
Background: Jasper is an AI copywriting and content generation platform geared towards marketing teams and businesses. Users can generate blog posts, ad copy, product descriptions, and more by inputting prompts, Jasper’s LLM-powered assistant then produces tailored content. Jasper serves a range of industries (healthcare, real estate, etc.) by speeding up content creation with consistent quality.
Monetization: Jasper uses a tiered subscription model priced per user (“seat”) with feature-based plans. For example, the Creator plan (for individual use) starts around $39/month per seat. Higher tiers like Pro or Business include more users and advanced capabilities (brand voice customization, SEO mode, collaboration tools, etc.) for larger orgs, often running in the tens of thousands per year for enterprise licenses. Pricing scales primarily by number of seats and feature needs, rather than usage.

Why this model: Jasper’s business customers integrate the tool into daily content workflows, so a per-seat subscription aligns with the value each user gets. Marketing teams prefer predictable costs and unlimited writing rather than worrying about per-output charges.
Jasper’s tiered plans let small teams start affordably and then expand. The high-end custom plans (ranging up to $70k/year for big enterprises) reflect the significant ROI large marketing orgs expect from AI-boosted content production. This subscription approach drives recurring revenue for Jasper while allowing customers to generate content freely to achieve their marketing outcomes.
2. Copy.ai: Freemium to Paid AI Writing Assistant
Background: Copy.ai provides an AI writing assistant with templates to generate marketing copy, social media posts, emails, blog sections, and more. It targets both individuals (creators, freelancers) and business teams looking to automate writing tasks. By 2025 the platform expanded collaboration features, allowing teams to co-edit content and maintain brand voice.
Monetization: Copy.ai employs a freemium model with usage-based limits that lead into paid plans. The Free Plan offers a taste, e.g. 2,000 words per month free (and a one-time 200 bonus credits for AI “Workflows”), enough for light use.
Serious users upgrade to Premium tiers: a Starter plan at ~$49/month (or ~20% off annually) for individuals, which unlocks unlimited chat interactions and the latest models, and an Advanced plan at ~$249/month that supports teams of up to 5 with 2,000 workflow credits and advanced features. Enterprise plans are available for larger organizations with custom pricing. Notably, Copy.ai also sells workflow credits, a form of usage-based pricing, so companies can pay for additional AI compute as they automate more processes.
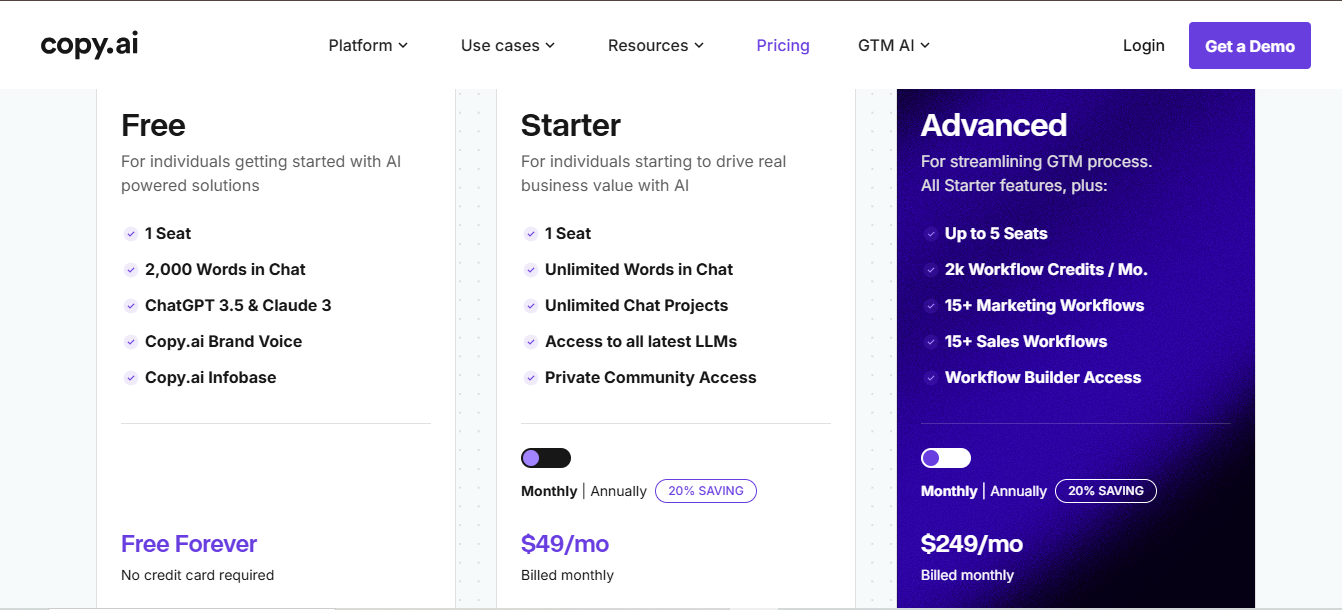
Why this model: This hybrid approach lets Copy.ai capture a broad user base. The free tier hooks individual users with basic needs (demonstrating outcomes like faster copy generation), while the subscription plans monetize power users on a monthly basis. Including usage credits in higher plans (and allowing top-ups) ensures revenue scales with heavier utilization, important because generating lots of content costs more compute.
For example, a marketing agency on the Advanced plan pays more but also gets more AI output (workflow runs) in return. This mix of recurring subscription plus usage-based add-ons aligns price to value: small users pay little or nothing, and heavy business users pay proportionally more as AI drives more of their content creation.
3. Writer: Enterprise AI Writing with Per-Seat Pricing
Background: Writer (writer.com) is an AI writing assistant tailored for enterprise teams who need to produce content consistent with their brand and communications guidelines. It offers custom large language models (like its Palmyra model family) that can enforce style guides, terminology, and factual accuracy for a company’s content. Writer is used in marketing, support, HR, and other departments to draft and edit text with AI while preserving an enterprise’s voice and standards.
Monetization: Writer uses a classic SaaS seat-license model with an enterprise twist. Smaller teams can start on a self-serve Starter plan at $39 per user monthly (or $29 when billed annually) for up to 20 users, this includes a generous allowance (e.g. ~50K AI-generated words per user) and core features.
Enterprises with bigger teams and custom needs negotiate Enterprise plans (custom pricing) that include unlimited usage, advanced security (SOC 2, HIPAA compliance), dedicated support, and even on-prem/private cloud deployment if needed. In practice, enterprise contracts often run in the five to six figures annually (one analysis showed a median of ~$32k/year for enterprise customers).

Why this model: Per-seat subscription pricing fits Writer’s enterprise focus because it aligns with how companies budget software (per user) and encourages organization-wide adoption. Every content creator or editor who uses Writer adds to the subscription, and those users can then generate as much AI content as they need (plans often have “unlimited” words for enterprise, removing usage anxiety).
This model also reflects outcome-based thinking: the value is tied to each employee using the tool to be more productive and on-brand, rather than counting each sentence generated. Writer’s annual billing options and enterprise customizations support long-term rollouts, which is key for an AI platform that often requires stakeholder trust (data privacy, brand integrity). Overall, the pricing strategy lowers the barrier for small teams to start (low per-user fee) and then grows as Writer proves its ROI across more users in a company.
4. Regie.ai: Outcome-Oriented Pricing for AI Sales Platform
Background: Regie.ai is a generative AI platform for sales teams, known as an “AI Sales Engagement” solution. It auto-generates sales outreach sequences (emails, LinkedIn messages, call scripts) and even provides AI agents for prospecting. Essentially, Regie aims to boost outbound sales productivity by automating content and outreach tasks with AI, driving more pipeline for businesses.
Monetization: Regie has a bold take on enterprise pricing, it emphasizes value and outcomes (meetings, pipeline) rather than charging simply for software access. In practice, Regie’s pricing is structured by the size of the sales team and additional AI capabilities needed. The core RegieOne platform is priced based on the number of prospecting sales reps, starting at $35,000 per year (for a base package).
This annual license includes use of the AI engagement platform for all those reps. On top of that, Regie offers add-ons like an AI-powered dialing system, which might cost +$20 per rep per month for basic dialing or up to $150/rep for advanced parallel dialing. All plans require talking to sales (no self-serve), and they pitch it as “paying for prospecting impact, not per-seat software.” In short, a mid-sized sales org might spend tens of thousands annually on Regie in exchange for AI-generated pipeline.
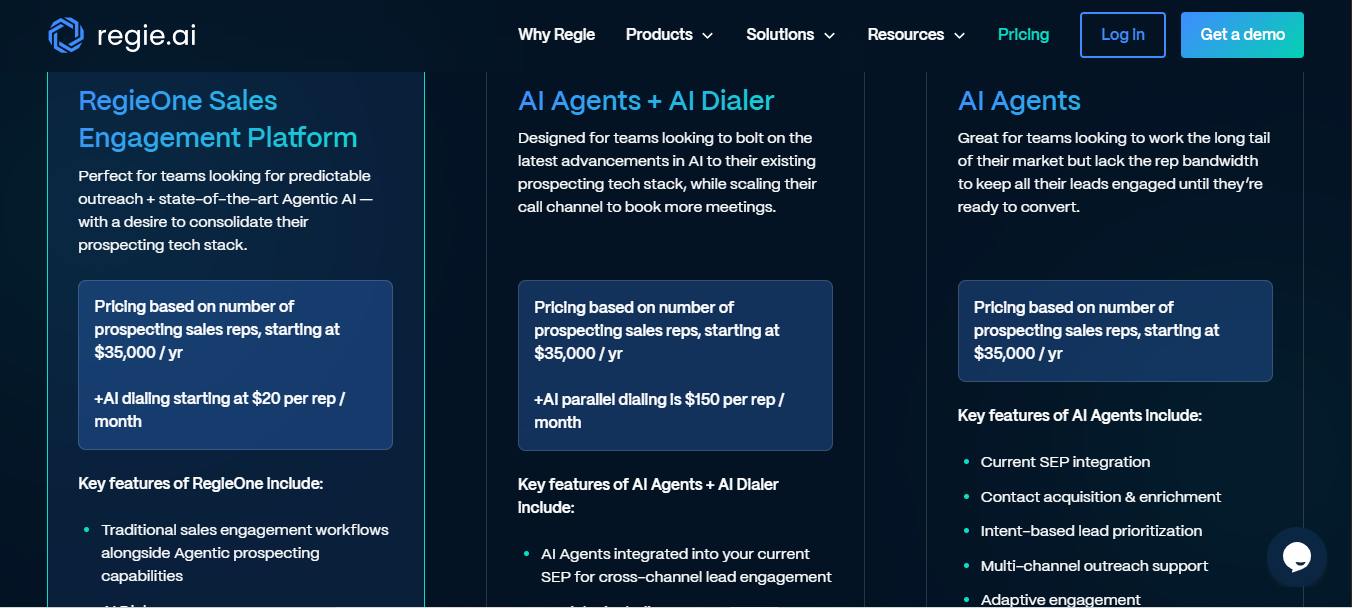
Why this model: This outcome-based hybrid pricing aligns with how enterprises evaluate sales tools, by ROI in pipeline and deals. By pricing “per rep” with a high annual minimum, Regie ties cost to the scale of the sales force (which correlates with potential pipeline generated), effectively charging for the capacity to drive results.
The messaging “value is in outcomes, not access” resonates with sales leaders: they’re willing to invest five or six figures if the AI sequences and calls lead to significantly more meetings booked. The usage-based component (dialer minutes per rep) adds a pay-for-what-you-use element for heavy phone users, but the bulk of the price is a fixed subscription. This ensures Regie gets a baseline revenue that scales with customer size, while also framing the purchase as an investment in revenue growth (making it easier for Regie’s champions to justify the budget). It’s a high-touch, high-value model well-suited to an enterprise product promising direct revenue outcomes.
5. Synthesia: Subscription Plans Tied to Video Length
Background: Synthesia is a platform for creating AI-generated videos. Users can type in text and choose an AI avatar/presenter, and Synthesia will produce a video with the avatar speaking the script in multiple languages. Businesses use it for training videos, marketing, how-to content, or personalized messages, eliminating the need for studios and actors. It’s popular for enterprises creating lots of internal videos (onboarding, tutorials) quickly.
Monetization: Synthesia offers a free trial and then tiered plans that primarily differ by the amount of video content you can generate. The Starter (Personal) plan is around $30 per month (or as low as $18/mo if paid yearly) and allows up to 10 minutes of video generation per month (approximately 120 minutes per year).
The next tier, Creator (Small Business) at roughly $89/month ($64/mo annually), increases the limit to 30 minutes of video per month (360 min/year) and unlocks features like custom avatars and API access. Above that, an Enterprise plan is offered with unlimited video minutes and many advanced features (branding, SSO, multiple team workspaces) at custom pricing. Essentially, Synthesia charges a subscription that includes a certain allotment of AI video output, with higher price points allowing more video minutes. Extra video credits can also be purchased if users exceed their plan, and large organizations negotiate custom rates.
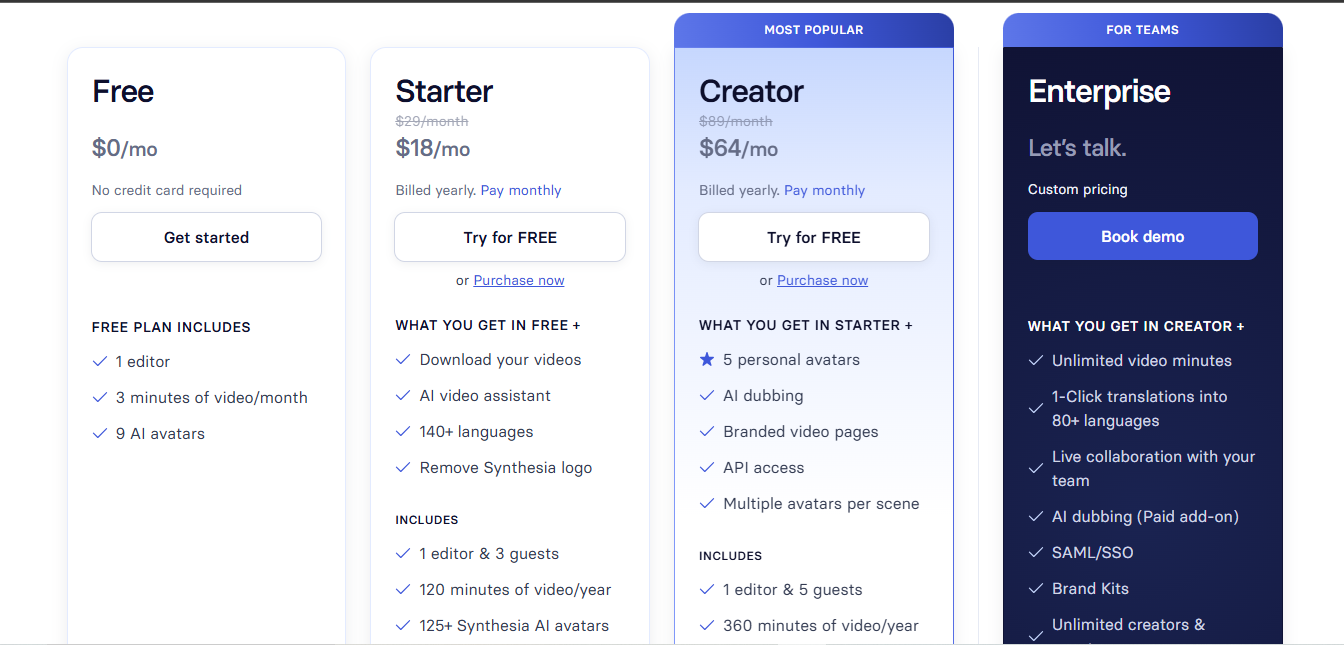
Why this model: Generating AI videos is computationally expensive, so tying pricing to video duration ensures the fee reflects usage (outcome) while still providing simplicity via tiers. Subscription bundles (e.g. pay $30 for ~10 minutes/month) give users predictable costs for a predictable output volume, this fits customers like training departments who plan a certain number of videos. It also anchors the value per video: for instance, 10 minutes of professional video content for $30 is very compelling compared to traditional video production costs.
The enterprise “unlimited” option at a higher price caters to companies that want to use AI video at scale without worrying about minute quotas, effectively offering a flat rate for potentially hundreds of videos (and pricing is set such that Synthesia still profits given typical use). By lowering unit costs at higher tiers, Synthesia’s model encourages customers to upgrade as their video needs grow. If you find value in AI videos, moving to the $89 tier gives 3x the content for about 2× the price, which is an outcome-driven upsell. Overall, this usage-aligned subscription ensures that those who create more videos (and presumably derive more business value from them, like more training or marketing reach) pay correspondingly more.
6. Runway: Credits and Plans for Generative Video Editing
Background: Runway is an AI creative suite known for its genAI video tools (like text-to-video and video editing models). Creators and media teams use Runway’s web platform to generate video content, apply AI effects, remove backgrounds, and so on. The company gained attention for its Gen-2 model that can generate short video clips from text prompts, appealing to filmmakers, designers, and even hobbyists looking to produce video content without traditional filming.
Monetization: Runway’s pricing blends freemium access with a usage-based credit system packaged into monthly plans. There’s a Free tier (free forever) that gives new users 125 credits one-time (worth about 25 seconds of generated video) so they can test the tools. Beyond that, paid plans include a monthly credit allowance plus additional features.
The Standard plan is about $12 per user per month (billed annually) and comes with 625 credits per month (roughly enough for ~50 seconds of high-end video or a few minutes of lower-resolution video). The Pro plan at $28 per user per month (annual) provides 2,250 credits per month (equating to several minutes of GenAI video generation) and unlocks all features.
There’s even an Unlimited plan (around $76/user/month, annual) that includes the Pro features and allows unlimited generations in a special “relaxed” mode. If users run out of credits, they can buy more à la carte or upgrade their plan. Enterprise customers can get custom plans with dedicated infrastructure and volume pricing.
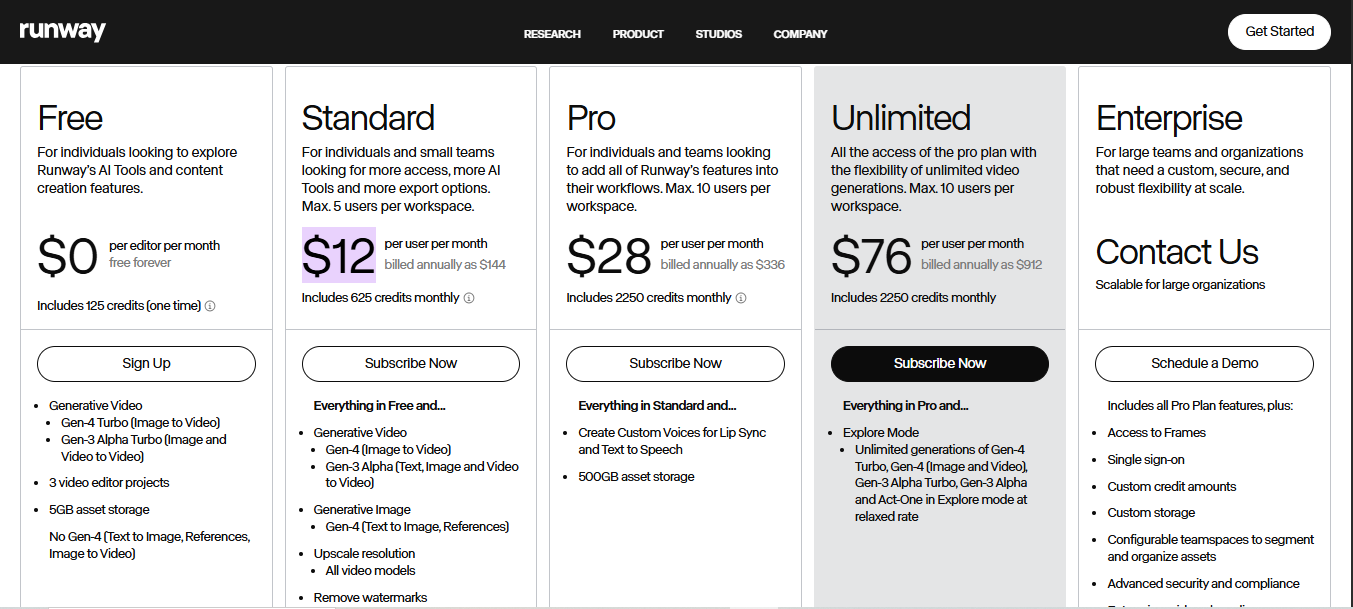
Why this model: Runway’s credits = compute approach directly ties pricing to the output (video or image seconds) consumed, which is an outcome-based metric for creative work. By bundling credits into monthly subscriptions, they ensure a baseline recurring revenue while accommodating different usage levels.
A casual creator might be fine on Standard ($12 for maybe a few short AI videos a month), whereas a professional studio will opt for Pro or Unlimited to get far more output. The per-user element also reflects the collaboration aspect, agencies with multiple editors pay per seat. The free tier is key for acquiring users by letting them see results (e.g. generate 500 images or a short clip) at no cost.
Importantly, Runway’s model encourages users to upgrade as they do more ambitious projects: if you start hitting your credit limit regularly (i.e. making longer or more frequent videos), it likely means you’re getting real value, at which point paying more for a higher plan makes sense. This aligns cost with the creative impact achieved.
The “Unlimited” option on a high-priced plan is interesting, it provides peace of mind for power users who don’t want to micromanage credits, while Runway can offer it because they throttle the generation speed in that mode (ensuring resources aren’t abused).
Overall, the mix of subscription + usage credits gives flexibility and fairness, making sure those who leverage AI to create lots of video (a valuable outcome, potentially saving thousands in production costs) pay a commensurate fee.
7. Fireflies.ai: Freemium with Tiered Per-Seat Plans for Meeting AI
Background: Fireflies.ai is an AI meeting assistant that joins virtual meetings (Zoom, Teams, etc.), transcribes the conversation, and generates summaries and action items. It essentially creates searchable meeting notes automatically. This tool is used by individuals and businesses to keep records of discussions and free participants from manual note-taking. It’s a productivity booster that leverages speech recognition and NLP to produce transcripts and insights from calls.
Monetization: Fireflies uses a classic freemium to paid upgrade model with pricing per user (seat) and increasingly rich features at higher tiers. The Free plan allows anyone to try it with limitations (e.g. limited AI summary capabilities and 800 minutes of storage per seat, and maybe a cap like 10 meetings a month).
Once users see the value, they can move to Pro at $10 per user per month (annual). Pro unlocks unlimited transcription and unlimited AI summaries for that user, with a larger storage quota (e.g. 8,000 minutes). Many small teams opt for the Business plan at $19 per user/month which includes everything in Pro plus unlimited storage and advanced analytics/features for team use. At the top, an Enterprise plan (around $39 per user/month) offers enterprise-grade controls, API access, SSO, and custom support.
All paid plans are significantly discounted if billed annually. Fireflies also offers a free trial and in some cases allows free users a limited number of “free meeting credits” (e.g. 10 meetings up to 30 min) before requiring upgrade.
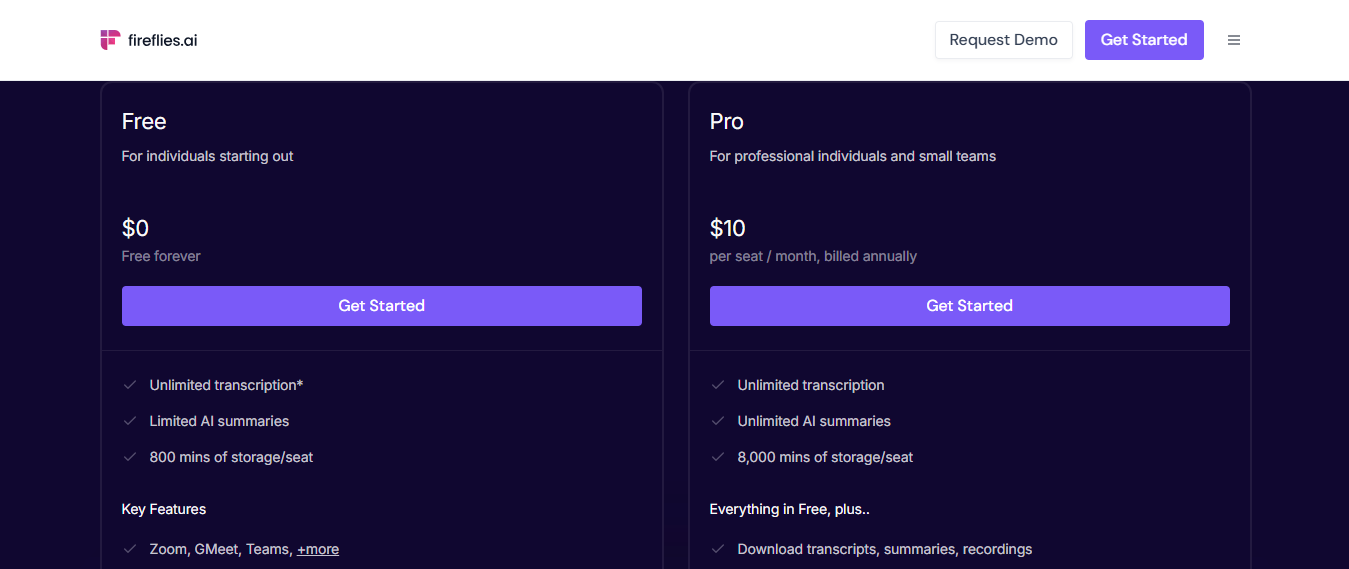
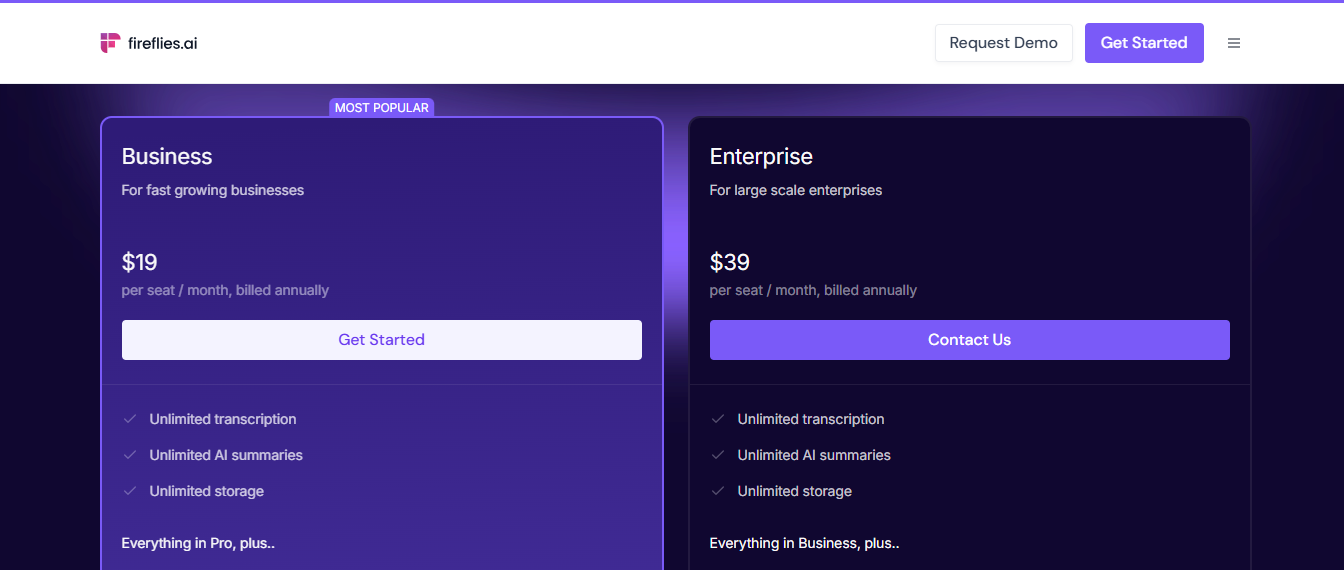
Why this model: This pricing structure maps well to user value and willingness to pay. Individuals can use Fireflies free for a few meetings to experience the outcome, accurate transcripts and summaries – which often is enough to convince them how much time it saves.
At that point, paying ~$10/month for unlimited usage is a no-brainer for professionals (the cost of one lunch for a tool that might save hours of writing minutes). The per-seat pricing means teams pay based on how many people actively benefit; for example, a sales team of 5 each paying $19/mo can record and analyze all client calls, a fraction of the cost of missing details that could close deals.
Fireflies’ decision to include “unlimited transcription” in paid tiers is outcome-driven: they don’t nickel-and-dime per minute or meeting, because they want users to fully adopt it for all meetings (the more meetings captured, the more value in having a searchable knowledge base). Instead, they differentiate by features and storage.
This flat usage approach on paid plans also signals confidence in their efficiency (transcription costs have dropped, allowing them to offer unlimited). The result is a low-friction, scalable model: as companies add more employees or hold more meetings, they simply add more user licenses, aligning with growth. The availability of higher-priced enterprise options with extra admin/control features also shows a monetization pathway as Fireflies’ customers mature, e.g. a startup might start on Pro, upgrade to Business as they grow, and eventually move to Enterprise as they require advanced security.
In summary, Fireflies’ freemium-per-seat model works because it converts the outcome (no more manual note-taking) into a modest recurring fee that feels proportional to the productivity gained.
8. Notion AI
Background: Notion is a popular all-in-one workspace app for note-taking, documentation, and project management. In early 2023, the company introduced Notion AI, an integrated generative AI assistant embedded within the Notion platform. This feature helps users draft content, brainstorm ideas, summarize notes, translate text, and answer questions directly inside their Notion pages. Notion AI is designed for the broad spectrum of Notion’s user base, from individual note-takers and students to enterprise teams, enabling them to leverage AI in their daily workflows without switching to a separate tool.
Monetization: Notion AI is offered as a paid add-on to Notion’s core plans (including the free tier). Initially, Notion provided a limited free trial (e.g. 20 free AI responses per user) to let users test the AI features. Beyond that trial, access to Notion’s AI requires a flat monthly subscription: $10 per member on a month-to-month basis, or $8 per member per month if billed annually. This add-on fee grants the user “unlimited” use of the AI features across their workspace, with no hard caps on the number of prompts or generations. In practice, Notion enforces a fair-use policy behind the scenes to prevent excessive usage from a single user (for example, very heavy users may be throttled if they generate an unusually high number of requests in a short time). However, typical users will never hit a visible limit, allowing them to integrate the AI heavily into their work without worrying about usage quotas. By monetizing via a per-user fee, Notion effectively layers a seat-based pricing model on its AI capabilities, mirroring how it already charges for the core product (seats in a workspace) rather than adopting a pay-per-use token or credit system.
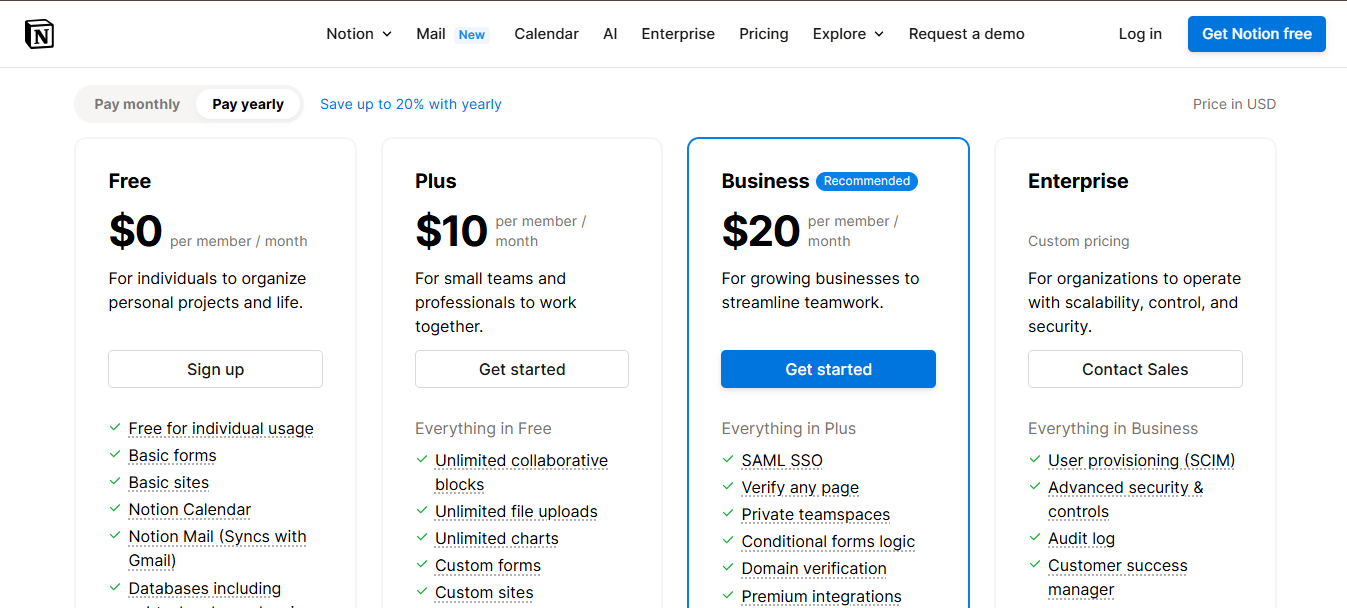
Why this model: Notion chose a seat-based add-on model for its AI in order to align with its existing SaaS pricing and maximize adoption of the feature. Charging a predictable per-user rate makes it simple for teams and individuals to understand costs and budget accordingly – a familiar approach that buyers are comfortable with. This predictable pricing lowers friction: users are encouraged to use the AI freely as needed, which increases the feature’s value, instead of holding back for fear of racking up fees.
Strategically, making AI an optional paid feature allows Notion to increase monetization per user (ARPU) without forcing it on everyone. Free or non-paying users can upgrade just for the AI benefits, and existing paying customers can add it if they find value, creating a new revenue stream on top of the core product. The flat fee also positions Notion AI competitively against standalone generative AI services, at roughly half the price of other premium AI tools (for example, Notion AI’s $10/user vs. $20 for ChatGPT Plus), it undercuts rivals while delivering the convenience of in-context AI.
By keeping pricing simple and tied to user seats, Notion leverages the “network effect” within organizations: if some team members start finding success with AI, it’s easy to justify enabling it for additional users at a known per-seat cost. Finally, the decision to forgo usage-based billing in favor of an all-you-can-use model reflects a strategic bet on driving engagement and retention: the more that users rely on Notion AI for their daily work, the more indispensable Notion as a whole becomes. The fair-use safeguards ensure that this unlimited usage promise is sustainable for the company, while most customers get a generous experience.
Overall, Notion’s pricing model for its AI add-on balances monetization and user experience, it captures value by charging for advanced functionality, yet maintains the straightforward, predictable economics that SaaS customers expect, thereby encouraging widespread adoption of the new AI features within its growing user community.
Segment Summary: Enterprise SaaS GenAI companies tend to charge recurring subscriptions per user or per company, often with unlimited core usage in those plans. This fits the enterprise preference for predictable costs and reflects that the outcome (better content, faster sales outreach, automated notes) is delivered continually, not as one-off transactions.
Many of these companies also emphasize free trials/freemium and tiered features to land users and then expand usage. Notably, a few (like Regie.ai) are experimenting with pricing that explicitly ties to business value (pipeline) or scale (per rep) rather than pure seat or compute metrics, showing an evolution toward outcome-driven pricing narratives even within a subscription framework.
Developer APIs: Generative AI Model Platforms
This next group of companies provides generative AI models and infrastructure via APIs or developer platforms. Essentially, they sell AI-as-a-service to developers and enterprises who want to build their own applications on top of large language models (LLMs) or other generative models. The common thread in this segment is usage-based pricing, customers pay for the volume of API calls or tokens (text characters) processed. This aligns with a very direct outcome: you pay for what you generate or analyze. Below we profile seven leading GenAI API providers and their pricing models:
9. OpenAI: Pay-As-You-Go for API, Plus Subscription for ChatGPT
Background: OpenAI is the most famous provider of large language models (like GPT-3.5 and GPT-4) and also the creator of ChatGPT. Developers can use OpenAI’s APIs to integrate generative text (and now image via DALL·E) into their own apps. Meanwhile, consumers and professionals interact with OpenAI’s tech through the ChatGPT interface. By 2023–2025, OpenAI serves a huge ecosystem ranging from individual tinkerers to Fortune 500 companies building on its models.
Monetization: OpenAI employs a dual model:
1. API usage billing
The OpenAI API is pay-as-you-go, charging based on the number of tokens (pieces of text) processed by the model. Essentially, each 1,000 tokens (about 750 words) of input or output has a price depending on the model’s complexity.
For instance, using the gpt-3.5-turbo model might cost around $0.002 per 1K tokens generated, while the more powerful GPT-4 can cost $0.03–$0.06 per 1K tokens (i.e. a few cents for a response). These rates are usage-based and no monthly minimum – developers only pay for what their users actually generate. This granular billing is often measured in fractions of a cent and summed up monthly. OpenAI also provides some free credit to new API users (e.g. $5 credit) to get them started.
2. ChatGPT subscriptions
In addition to API, OpenAI monetizes ChatGPT’s web interface via a premium subscription called ChatGPT Plus at $20/month. Free users can use ChatGPT (with GPT-3.5) at no cost, but Plus subscribers get benefits like access to the more advanced GPT-4 model, faster response times, and priority access even during peak times. Millions of users have opted for this $20/mo plan, making it a significant revenue stream. Another subscription they offer for Pro users is at $200/month that includes everything in plus, with some additional features like:
- Unlimited access to all reasoning models and GPT-4o
- Unlimited access to advanced voice
- Extended access to deep research, which conducts multi-step online research for complex tasks
- Access to research previews of GPT-4.5 and Operator
- Access to o1 pro mode, which uses more compute for the best answers to the hardest questions
- Extended access to Sora video generation
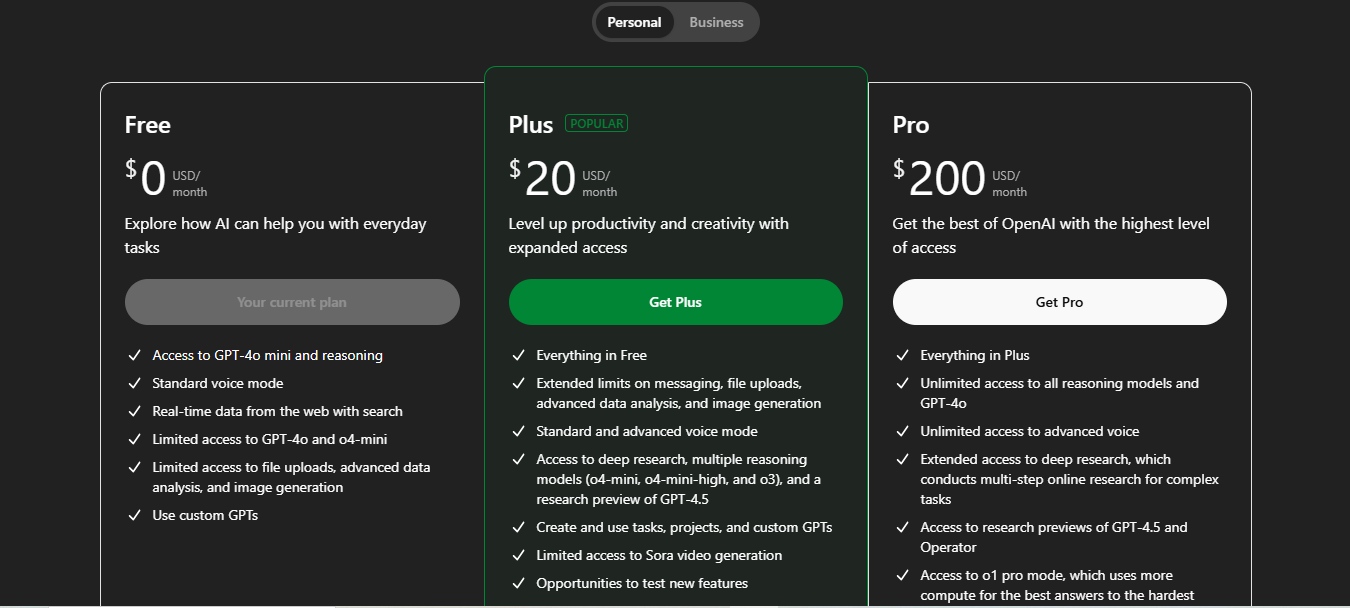
Why this model: OpenAI’s approach reflects two distinct value propositions. For developers, the usage-based API pricing is ideal – it’s transparent and scalable, allowing developers to start small (even free) and only incur costs as their app gains usage. This lowers the barrier to integrating AI, which helped OpenAI achieve widespread adoption. It also ties cost directly to outcome delivered: if an app makes 1,000 API calls generating answers or content, the developer pays for exactly that amount of AI service (in essence, paying only when the AI is actually creating value in their app).
The token-based model also encourages efficient prompt design, which aligns with OpenAI’s need to manage compute load. On the ChatGPT consumer side, a low monthly subscription has proven extremely effective. The $20 price point is low enough that enthusiasts, students, and professionals worldwide find it worth paying for a much more powerful AI experience (GPT-4) and reliability.
This recurring revenue from ChatGPT Plus essentially monetizes heavy users who value the AI’s output for work or study – an outcome worth far more than $20 (like writing code, summarizing research, brainstorming ideas). By keeping a free tier for ChatGPT, OpenAI also maintains a broad user base (important for feedback and goodwill) while converting a fraction of them to paid. In short, usage fees on the API capture value from the developer ecosystem (and big enterprise API users can rack up substantial monthly bills), and the flat subscription on ChatGPT captures value from individuals at scale.
It’s a powerful combination that turned OpenAI into a revenue-generating leader in GenAI.
10. Anthropic: Usage-Based Pricing with Volume Options (Claude)
Background: Anthropic is an AI startup known for its large language model Claude, a competitor to OpenAI’s GPT series. Claude is offered via an API (and a chat interface) and is touted for its ability to handle very large context windows (long documents) and for its constitutional AI approach (safety). Developers and enterprises can use Claude for tasks similar to GPT, from summarization and Q&A to content generation, and Anthropic continuously improves model versions (Claude Instant, Claude 2, etc.). In 2023, Anthropic received major investments (including from Google) and became a key player providing foundation models as a service.
Monetization: Anthropic monetizes Claude through a combination of usage-based API pricing and tiered subscription plans for individuals and teams, reflecting a dual strategy: developer-first APIs and productivity-oriented consumer access.
For API access, Anthropic follows a usage-based model similar to OpenAI, charging per thousand tokens of input and output. As of their latest pricing:
- Claude 3 Opus: $15.00 per 1M input tokens / $75.00 per 1M output tokens
- Claude 3 Sonnet: $3.00 per 1M input / $15.00 per 1M output
- Claude 3 Haiku: $0.25 per 1M input / $1.25 per 1M output
In practical terms, generating ~1,000 words with Claude Opus might cost between 5-7 cents, depending on complexity and formatting. Enterprise users can also opt for reserved throughput capacity, buying guaranteed token-per-minute rates at fixed hourly pricing, which is ideal for high-scale deployments with strict latency and performance requirements. Access via Amazon Bedrock often comes with slight discounts and integration benefits.
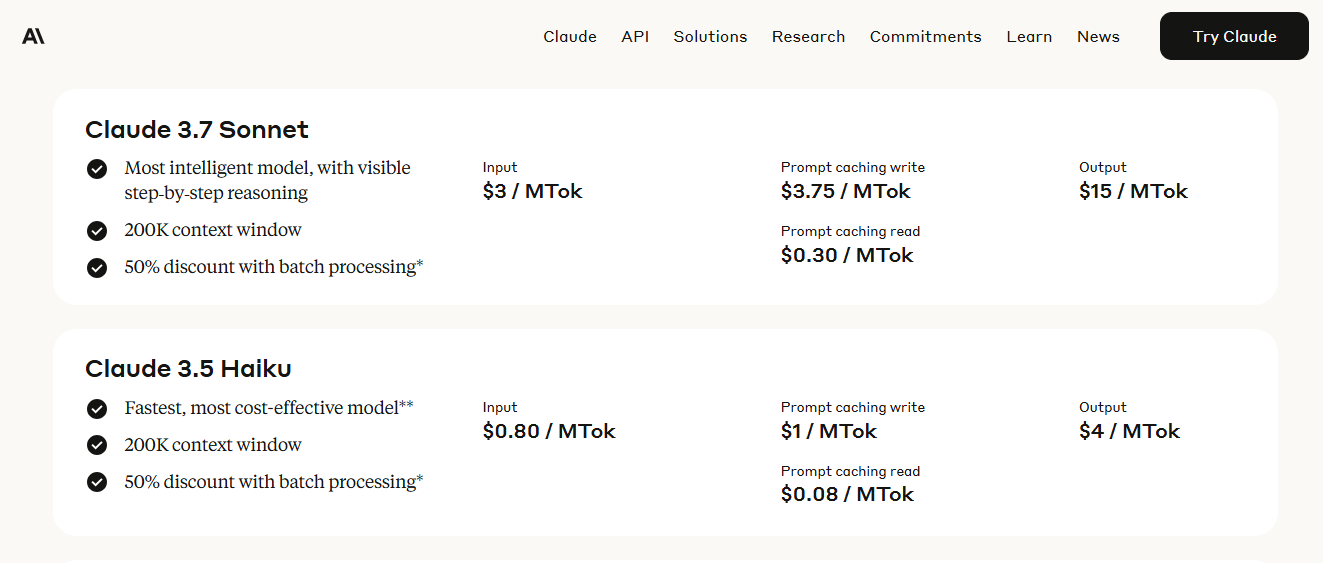

On the consumer side, Anthropic offers web and mobile chat interfaces through three subscription tiers:
- Free: $0/month: Includes access to basic Claude features on web and mobile.
- Pro: $20/month (or $17/month with annual billing): Adds extended usage, web search, Google Workspace integrations, and access to more Claude models.
- Max: Starts at $100/month: Unlocks higher limits, priority access, Claude Code in terminal, advanced research tools, and early feature access.

Unlike OpenAI, Anthropic hasn’t released a low-cost standalone chatbot product. Instead, it targets power users, developers, and enterprises, leaning heavily on premium productivity features and scalable API access as its core monetization levers.
Why this model: Being a developer-focused company, Anthropic adopted pay-as-you-go pricing to lower adoption friction. Clients pay only for what they use, which is straightforward and developer-friendly (no seats or subscriptions to negotiate). This also reflects the cost structure: running these models consumes GPU time, so charging by tokens ensures revenue aligns with compute consumed. By making the pricing proportional to usage, Anthropic can serve a range of customers, from a startup that might spend just a few dollars a month on Claude API, to a large enterprise that spends thousands or more.
When customers scale up significantly, Anthropic’s provisioned throughput option essentially converts the model into a subscription-like utility (buying a dedicated slice of the model’s capacity per hour). This is outcome-driven for big users: if you know you need a high volume of Claude outputs (say, because you’re doing AI customer support at massive scale), committing to a throughput ensures you can serve that outcome reliably and often comes at a bulk discount.
In summary, Anthropic’s pricing is designed to compete on transparency and flexibility, by letting users pay by the token, they directly compete with OpenAI on cost (e.g. Claude 2’s input costs are about 40% lower than GPT-4’s for some usages) and try to attract businesses that are sensitive to price or need model flexibility.
The usage model also reinforces that customers are paying per AI-generated result, making it easier to measure ROI (for instance, cost per successful answer or per 1,000 docs summarized). This usage alignment is expected in the API space and Anthropic follows it to position Claude as an on-demand AI service.
11. Cohere: Token-Based Pricing with Enterprise Plans
Background: Cohere is a Toronto-based AI company offering large language models and NLP services via API. Cohere’s platform provides not just text generation (similar to GPT) but also embeddings for search, classification, summarization, and reranking. It has positioned itself as an enterprise-friendly, secure AI platform, often highlighting data privacy (not training on client data) and multi-language capabilities. Cohere’s models power applications like content generation, customer support bots, and analytics in multiple languages.
Monetization: Cohere’s pricing is usage-based, measured in tokens or specific calls, with different endpoints priced separately to reflect their computational load. They also have free tiers and volume discounts for enterprise. For example:
Generative Models (Command Series)
Cohere offers a range of generative models suited to different needs—from high-performance agents to lightweight apps:
- Command A (newest and most powerful):
$2.50 per 1M input tokens- $10.00 per 1M output tokens
- Best for agentic AI, multilingual applications, and human-like responses.
- Command R+:
- $2.50 per 1M input tokens
- $10.00 per 1M output tokens
- Enterprise-grade model tuned for real-world performance and scale.
- Command R:
- $0.15 per 1M input tokens
- $0.60 per 1M output tokens
- Optimized for long-context tasks like retrieval-augmented generation (RAG) and external API calling.
- Command R (Fine-tuned):
- $0.30 per 1M input tokens
- $1.20 per 1M output tokens
- Tailored for niche or domain-specific tasks.
- Command R7B (smallest and fastest):
- $0.0375 per 1M input tokens
- $0.15 per 1M output tokens
- Ideal for lightweight applications where speed and cost matter most.
Total cost is based on the sum of input and output tokens processed per request.
Retrieval Models
- Rerank 3.5:
- $2.00 per 1,000 search queries
- Each query can rank up to 100 documents. Documents over 500 tokens (including query length) are split and billed as multiple chunks.
- Embed 4:
- $0.12 per 1M text tokens
- $0.47 per 1M image tokens
- Multimodal model supporting semantic embeddings for both text and images, ideal for search and RAG pipelines.
Other API Endpoints
- Classify:
- $0.20 per 1,000 items (or $0.0002 each)
- Suitable for scalable classification and labeling tasks.
Pricing aligns with generative models (e.g., Command A or R), depending on the model used.
- Training:
- $3.00 per 1M tokens
- For fine-tuning generative models with custom data.
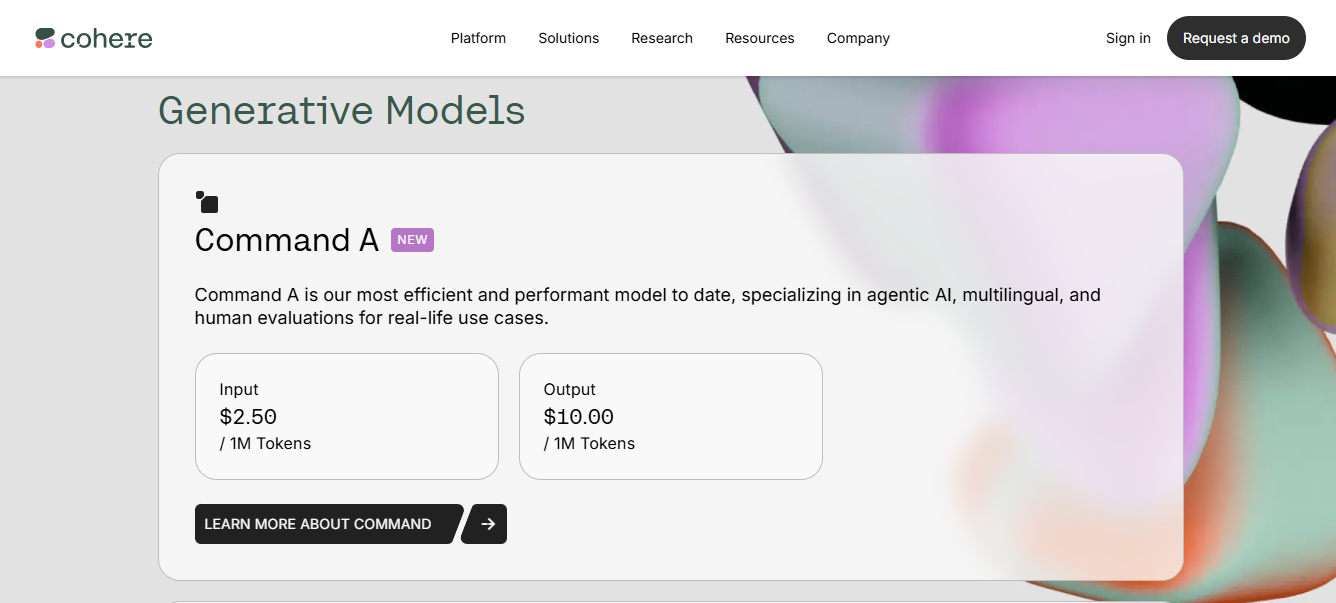
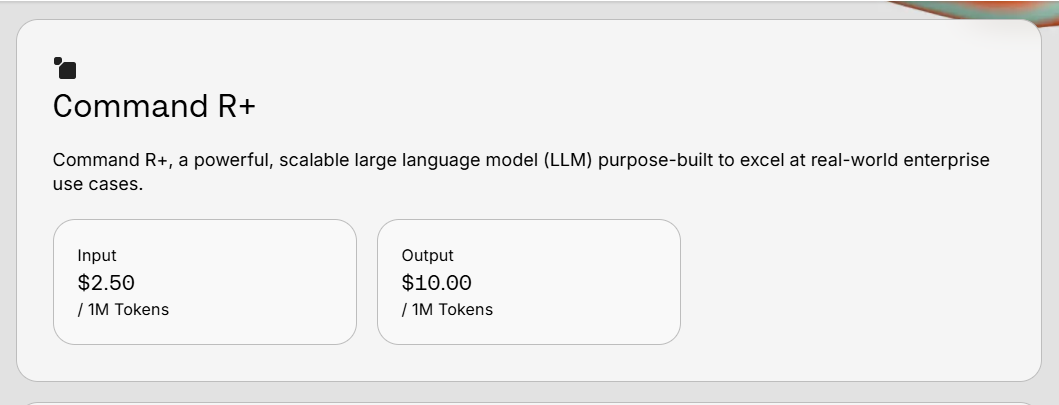


Cohere essentially unbundles each AI service with its own metric and price. They provide a free trial API key with rate limits (for instance, 5,000 generations per month free) to let developers experiment. For enterprise customers, Cohere offers custom pricing, private deployments, and likely subscription contracts that include a committed usage or monthly minimum.
Why this model: Cohere’s per-token pricing for its core endpoints shows it’s aligning with market norms (developers are used to thinking in tokens thanks to OpenAI). This granular model is attractive to the technical audience, “$15 per 1M tokens” comes across as cost-effective for content generation, and indeed Cohere often pitches itself as a cheaper alternative to the big players for certain use cases. Usage pricing also makes it modular: customers can choose to use just the Embed API or just Generate and pay for that alone, which aligns with the outcomes they need (e.g. a company might only need embeddings for search enhancement, so they pay $0.40/M tokens for that outcome, which is very affordable). This granular charging ensures neither party overpays, the customer isn’t forced into a big bundle, and Cohere is compensated in proportion to how heavily its models are used.
That said, Cohere recognizes enterprise buyers often prefer packages, so likely their large deals involve a committed spend for a bundle of usage (perhaps a flat annual fee that includes up to X million tokens, etc.). This hybrid approach (usage metrics under the hood, but enterprise license deals on top) is common in B2B AI, it provides flexibility for small users and predictability for big users.
The rationale is that if Cohere’s models deliver value like automating email writing or analyzing support tickets, the customer will scale up usage, and Cohere’s revenue will scale in tandem. Cohere’s published pricing also highlights the specific tasks (Generate vs Classify), which helps business users understand costs per type of outcome (e.g. “about $0.0002 per classification” frames the cost per prediction). This clarity is part of Cohere’s enterprise-friendly POV, they emphasize transparent, “no surprise” pricing to reduce uncertainty. In effect, Cohere’s model matches the utility computing paradigm: AI power is a utility charged by consumption, which is appealing for companies who want to avoid large upfront fees and only pay when AI is actually being applied to their business problems.
12. AI21 Labs: Pay per Text Generated (APIs for Jurassic, etc.)
Background: AI21 Labs is an AI company behind the Jurassic-2 family of language models and products like Wordtune. They offer AI-as-a-service for text generation and comprehension via their platform AI21 Studio. AI21’s models support tasks like writing assistance, summarization, and reading comprehension, and they often tout competitive performance on par with other big models. AI21 is also known for a focus on multilingual capabilities and has partnerships (their models are available through Amazon Bedrock, for example).
Monetization: AI21 Studio uses a usage-based pricing model very much like OpenAI’s. They price by characters or tokens processed, depending on model size:
- For instance, their smallest model Jamba (Jurassic) Mini is extremely cheap – on the order of $0.20 per 1M input tokens and $0.40 per 1M output tokens (that’s $0.0002 per thousand input tokens, practically negligible, and $0.0004 per thousand output tokens). This allows developers to use basic models for pennies.
- Larger, more powerful models like Jamba Large (or Jurassic-2 Ultra) cost more – e.g. about $2 per 1M input and $8 per 1M output. This might translate to ~$0.008 per 1K output tokens (0.8¢ for ~750 words), which is still quite cost-effective at scale.
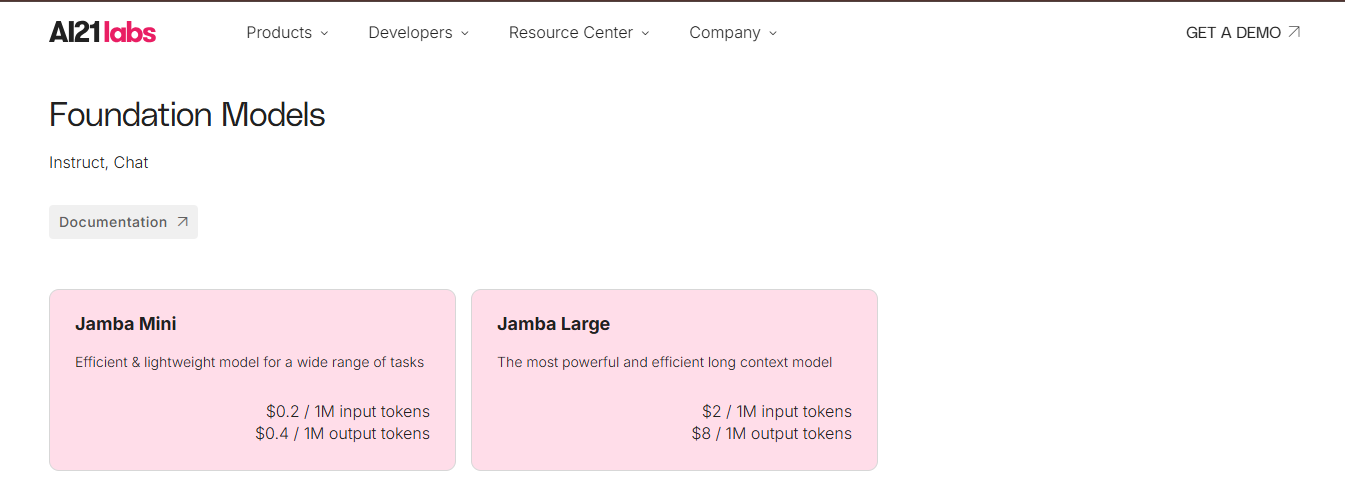
- They offer a free trial credit of $10 for new users, and all usage is pay-go beyond that.
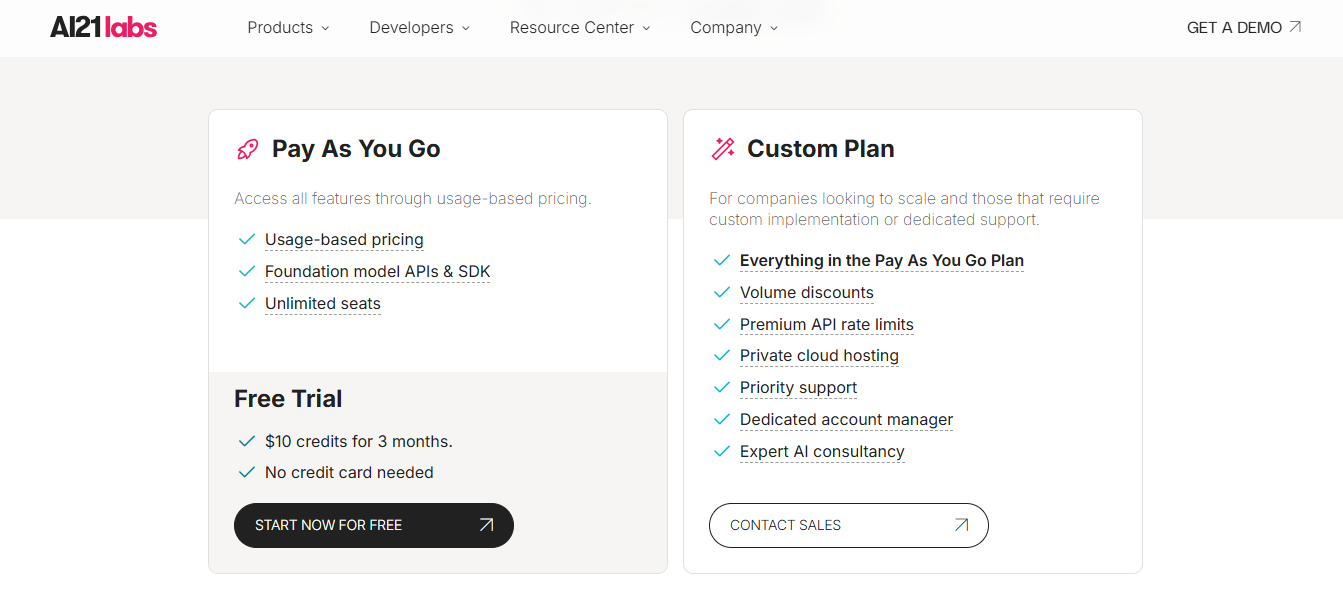
- AI21 also has a custom enterprise plan option: companies can get volume discounts, private model deployments, or reserved capacity by contacting sales. They effectively have two buckets: Pay-as-you-go (with “unlimited seats” – anyone in your org can use the API under one billing account), and Custom Plan for enterprises needing SLAs, support, and maybe flat pricing agreements.
Why this model: AI21 Labs is competing in a landscape dominated by bigger players, so they leverage transparent, lower pricing to attract developers and businesses. By charging by input/output tokens, they let customers directly compare costs to others. For example, at $8 per 1M output tokens for their large model, they undercut GPT-3.5 and GPT-4 on many tasks, making AI21 appealing for cost-sensitive deployments. This usage-based model also helps AI21 showcase that they reduce “unnecessary spend” with fine-grained pricing. Essentially, if a customer only needs a lightweight model, they pay incredibly low rates; if they need the big model’s quality, they pay more per token, the pricing follows the value delivered.
AI21’s free credits and no monthly fee approach make it easy to try (outcome-driven adoption: prove the quality, then start paying as you scale usage). As users integrate AI21’s models into their applications (e.g. an app that paraphrases text or answers questions), the costs scale in proportion to how many end-users use those features. This is economically efficient for the customer: their cost of AI will roughly track their user base or usage intensity, which usually correlates with their own revenue. In other words, AI21 gets to “share in the success” of its customers’ AI-powered features by charging per use.
For enterprise clients who might want a steadier arrangement, AI21’s custom plans likely allow committing to a certain spend or throughput for a discount (common approach). But under the hood even those deals are based on an estimated usage – they might say, for example, “we’ll commit to 50 billion characters a month for a flat $Xk fee”. The reasoning is that large enterprises integrating AI (like in an internal knowledge base or customer service system) want to avoid variable bills, so AI21 can accommodate volume pricing while still fundamentally measuring the usage. This way AI21 can appeal to both the developer audience (with self-serve, metered pricing) and the enterprise procurement (with negotiable contracts). In summary, the pay-per-text model of AI21 aligns cost to amount of content processed/generated, which is the core outcome their models provide. It’s simple, which fosters trust – users know exactly how much they pay for a given AI workload – and it encourages broader usage of AI21’s services as being potentially more cost-effective than the competition.
13. Stability AI: Credit System for Image Generation
Background: Stability AI is the company behind Stable Diffusion, the popular open-source image generation model. While Stability released models openly, they also operate DreamStudio – a web app and API for generating images (and soon other media) in the cloud without needing your own GPU. Stability AI is thus in the GenAI business by providing both open models and a hosted service for convenience. Their primary “product” is compute time for image (or art) generation using their models.
Monetization: Stability AI’s DreamStudio uses a credit-based usage model. Users purchase credits with real money, and each image generation or operation costs a certain number of credits based on its computational expense (resolution, steps, etc.). The baseline is 1 credit = $0.01 (one cent). By default, generating an average image (e.g. 512×512, 50 inference steps) used to cost 1 credit (=$0.01), but in late 2023 they drastically reduced prices – now an image at default settings might cost only 0.2 credits. In practice, Stability might sell packages like $10 for 1,000 credits (roughly 5,000 images at default settings after the price drop). New users get some free credits (e.g. 100 credits free, enough for ~500 images) to try it out. There are no monthly subscription fees, it’s purely pay-per-use by buying credit bundles.
For its API (Stability’s developer platform), the same credit system applies: an API call to generate an image deducts credits from your balance. If users need more, they purchase more credits. The model is very straightforward: essentially $0.01 = 1 standard generation was the old baseline, now even cheaper at $0.002 per image for default quality. Stability AI thus monetizes the compute behind Stable Diffusion, even though the model weights are free – what users pay for is the convenience and speed of running it on Stability’s servers (and access to upgraded proprietary versions like SDXL).
Why this model: This microtransaction-like pricing fits the product and audience. Many DreamStudio users are artists, designers, or hobbyists who might not generate images every single day – a subscription might turn them away, but a pay-per-image model means they can spend as little or as much as they want. It feels like buying tokens at an arcade: you only pay when you use it. By heavily dropping the credit cost per image (80% reduction as noted), Stability aligned price with the reality that image gen is getting cheaper (due to more efficient models and competition). Their goal is likely to undercut closed competitors (like Midjourney or DALL·E) on cost. For example, DALL·E 3 via OpenAI might effectively cost about 13 cents per image (if using GPT-4 to generate it), whereas Stable Diffusion via DreamStudio now costs ~$0.002 – essentially nearly free in comparison. Stability can do this because Stable Diffusion is open-source and can be run on consumer GPUs; they monetize at volume with thin margins per image, but a huge user base.
The credit system is also easily understandable: users see their credits decreasing with each image and know when to buy more. This is directly tied to outcome – each credit yields an image (or multiple images after the price update), so users can evaluate if those images are worth the spend. Moreover, this model is on-demand: enterprises or devs using the API can scale usage up or down without committing to a contract. If a marketing campaign needs 10,000 AI-generated visuals one month, they might spend $100 in credits that month and maybe much less the next. Stability only earns when its service is actively used, which is fair and efficient.
One downside of pure usage models can be revenue predictability for the company, but Stability likely addresses that by selling credits in chunks (getting cash upfront) and relying on consistent user demand. They may introduce subscriptions for heavy users later (like a plan that gives unlimited or a fixed number of generations per month for a flat fee), but at the time, the focus on low-cost, on-demand credits is part of their strategy to attract a large community. The bet is that making generative art essentially ultra-affordable will drive adoption, and even if each user spends only a few dollars, millions of users can add up. It also strengthens the Stable Diffusion ecosystem – more usage means more feedback and improvement. In short, Stability AI’s pricing is all about minimizing friction and maximizing scale: by charging tiny amounts per image and letting users pay only for what they need, they ensure anyone who has an “outcome” of a cool image in mind can afford to realize it.
14. Google (Vertex AI / PaLM API): Cloud Usage Pricing with Free Tier
Background: Google entered the GenAI API space by offering models like PaLM 2 and other generative services via its Google Cloud Vertex AI platform. While Google’s own chatbot (Bard) was free as of 2023, the Google Cloud GenAI APIs (text, chat, and embedding models) are how Google monetizes its AI models for businesses. Essentially, Google provides these models as another service in its cloud, similar to how they offer compute or storage.
Monetization: Google’s Vertex AI model endpoints follow a usage-based pricing model integrated into Google Cloud billing. They charge by units like characters or tokens. For example, the PaLM 2 text model was reported to be priced by the character of input/output during its preview. While the exact rates shifted as they rolled out new models (and now Gemini is coming), an illustrative pricing was something like: for 1 million characters generated, you pay a certain rate (perhaps a few dollars). Google also often provides a generous free tier during previews – e.g. free access up to certain limits (some reports said the PaLM API was free in trial period). Once billing starts, it’s purely pay-per-use, with charges appearing on the Cloud invoice just like any other API calls.
Google cloud customers can also negotiate enterprise agreements, so big clients might have committed spends that include Vertex AI usage. But for most, Google’s documentation lists prices per unit of text or per 1000 tokens. One hypothetical example: say Google priced PaLM 2 at $3 per million input characters and $6 per million output characters (just as an illustration) – this would equate to roughly $0.004 per 1K characters of output (~0.4¢ for 1000 characters). Actual numbers aside, the structure is “pay by volume of text” similar to competitors. There’s no separate subscription for the GenAI API; it’s a metered cloud service. Bard (the chat app) is free and effectively subsidizes learning and data, whereas the API is the paid product for developers.
Why this model: Google is essentially treating generative AI like a cloud utility. This is logical because their target customers are developers and enterprises already using Google Cloud services – these customers expect on-demand scaling and to be billed for exactly what they consume. By folding AI into that model, Google makes it easy for a Cloud customer to experiment and then ramp up usage without a new procurement process. The free quotas/trials encourage trying outcomes first – e.g. a developer can test generating some summaries or code with PaLM API at no cost, see the outcome, then decide to use it more. When they do use it heavily, charges accrue in a pay-as-you-go manner, which fits cloud budgets (many companies prefer OPEX that scales with usage vs. fixed upfront cost).
Also, Google likely benchmarked competitors and knew they needed to be cost-competitive or at least in the same ballpark. Usage pricing allows them to compete on a per-output cost basis. If Google’s models are very efficient or tuned, they might price them slightly lower per token to entice users away from OpenAI. Additionally, Google’s enterprise clients can leverage committed use discounts – e.g. commit to spend $X on Vertex AI and get a discount, similar to how they handle BigQuery or Compute Engine. This encourages larger deals while still fundamentally metering usage.
In essence, Google’s pricing strategy here is all about integration and scale: it leverages the existing cloud consumption model which is outcome-aligned (you pay for what you use, be it storage GB, compute hours, or AI tokens). This ensures that if a company uses Google’s GenAI to, say, generate millions of product descriptions (the outcome), the cost is proportional to that scale of outcome. There’s no separate seat license or subscription to worry about – just an incremental cost line-item on their cloud bill. The familiarity of this is a selling point. And as with others, if the AI delivers significant value (e.g. automating work that would have cost a lot in labor), the usage fees can be easily justified.
15. Amazon Bedrock: Flexible Consumption of Multiple Models
Background: Amazon Bedrock is AWS’s fully managed service for accessing multiple foundation models (from providers like Anthropic, AI21, Stability, and Amazon’s own Titan models) through one API. Launched in 2023, Bedrock allows AWS customers to build generative AI applications without dealing with model hosting – they can call models like Claude or Jurassic via the Bedrock API and pay through their AWS account. It’s part of AWS’s strategy to offer a one-stop shop for GenAI on the cloud.
Monetization: Bedrock’s pricing model is usage-based, varying per model, much like a marketplace. AWS essentially passes on the model inference costs, often with a slight premium for the managed convenience. For instance, AWS’s pricing for Anthropic Claude 2 was about $0.008 per 1K input tokens and $0.024 per 1K output tokens (similar to Anthropic’s direct pricing, possibly a tad higher). AI21’s Jurassic models on Bedrock have listed prices (e.g. Jurassic-2 Ultra around $0.0188 per 1K tokens). These are charged in API units on your AWS bill. There are no upfront fees to use Bedrock; you only pay when you invoke model endpoints. AWS provides some free tier usage for Bedrock during preview (like a limited number of requests free).
Because Bedrock is part of AWS, enterprise customers can negotiate enterprise discount programs that include Bedrock usage, or use committed AWS spend to cover it. Additionally, AWS offers features like Provisioned Throughput for some Bedrock model usage – meaning a customer can reserve capacity (at a fixed hourly rate) if they need consistent high-volume usage, akin to how they reserve instances for steady workloads.
Why this model: Amazon’s aim is to make adopting generative AI as easy and flexible as any other cloud service. By aligning pricing with usage, AWS ensures customers only pay for actual inference calls, which removes a barrier to entry. An AWS client can try out, for example, 100 calls to various models and see which one’s output best fits their needs, incurring maybe just a few cents or dollars total – effectively paying per outcome tested. This smorgasbord approach (multiple models) under a single pay-per-call scheme is powerful: it abstracts the complexity and lets the best model win on quality and cost.
From AWS’s perspective, usage-based billing is standard – all their services (Lambda, SageMaker, etc.) charge by request, duration, data processed, etc. Bedrock simply extends this to AI models. This “choose your model, pay per use” model is outcome-centric because it treats each model invocation as delivering a unit of value (an answer, a piece of content) and assigns a price to it. If customers get significant value, they’ll call more and AWS’s revenue increases correspondingly; if not, they won’t call and won’t pay much. This dynamic aligns AWS’s incentives with delivering high-performing models on Bedrock – if one model is too expensive for its quality, customers will switch to another (all within Bedrock).
It’s also noteworthy that Amazon, like Google, likely isn’t trying to profit heavily per API call initially – they want to gather enterprise workloads on their platform, then rely on volume. By making pricing competitive and usage-based, they reduce friction to experimenting with and adopting GenAI in enterprise workflows. Additionally, many AWS enterprise customers will prefer consolidated billing – Bedrock’s model means their AI usage just shows up as another line item, making accounting simpler (compared to, say, signing a new contract with an AI startup).
In summary, Amazon Bedrock’s pricing exemplifies flexibility and customer choice: it allows paying only for the outcomes (model inferences) you actually use, it lets you dynamically shift between models without financial penalty, and it integrates with existing cloud spending patterns. This encourages broader usage of generative AI, as companies can start small, find their use cases, and then scale confidently knowing costs will scale linearly with their usage.
Segment Summary: Developer-facing GenAI companies overwhelmingly embrace consumption-based pricing. Charging per token, character, or API call aligns with how developers consume these services – on demand and scalable. It ties cost directly to the AI output generated or processed, ensuring that if a model delivers a lot of value (through heavy usage), the provider earns commensurately. We see slight variations: some, like Cohere, price different endpoints separately (reflecting outcome complexity), while others, like OpenAI and Anthropic, simply charge by tokens irrespective of use case. In all cases, no long-term commitment is required to start, lowering risk for users. Enterprise deals in this space often involve volume discounts or reserved capacity (a hybrid of usage and subscription) once usage becomes predictable and high. The overall trend is that GenAI APIs are becoming a commodity utility – priced transparently, with competition driving costs down (e.g. Stability’s aggressive low pricing, or open-source models like MosaicML’s MPT being offered at a fraction of OpenAI’s cost). This benefits developers and ultimately end-users, as generative AI features get integrated into all sorts of products under sustainable cost structures.
Consumer AI Tools: Subscriptions and Freemium at Scale
This category includes AI-powered applications and services aimed directly at consumers or prosumers – think individuals, creators, students, or casual users. These tools often went viral or gained mass adoption (e.g. AI avatar apps, chat companions, image generators). The monetization here leans on models familiar in consumer software: freemium with in-app purchases, monthly subscriptions for premium features, and sometimes one-time payments. The goal is to convert a fraction of a large user base into paying users by offering enhanced outcomes (faster responses, higher quality outputs, exclusive features, unlimited usage, etc.). Let’s look at seven popular consumer-facing GenAI products and their monetization strategies:
16. Character.AI: Freemium Chatbots with Optional Subscription
Background: Character.AI is an AI chatbot platform where users can chat with a multitude of AI “characters” – from historical figures to fictional personas or even original characters created by the community. It’s used largely for entertainment, companionship, and exploration of AI personalities. The service gained a huge user base (especially among younger users) by offering open-ended, creative conversations for free.
Monetization: For most of its existence, Character.AI was free-to-use with unlimited messaging. In 2023, to generate revenue, the company introduced Character.AI Plus (c.ai+), a premium subscription at $9.99 per month.
This subscription gives paying users several perks:
- Faster response times from the AI (free users might wait in a queue during peak times, while Plus users get priority).
- Priority access when the service is busy (ensuring the service remains available even at high load).
- Early access to new features as they roll out. At launch, all the core features (unlimited chats, custom character creation) remained free, and the company explicitly stated it would continue offering free unlimited messaging for all users. The c.ai+ subscription is thus pitched as a way to “supercharge your experience” if you’re an avid user, rather than a necessity. There is no pay-per-chat or one-time purchase; it’s a classic monthly subscription for power users. Character.AI does not show ads as of 2025, so this subscription is the primary monetization.
Why this model: Character.AI’s user base runs into the tens of millions, many of whom are teens or casual users who might not pay at all. The freemium approach maximizes reach and engagement – it keeps the community large (which also helps the platform since users create and fine-tune character personas). By not paywalling essential features, Character.AI ensures that network effects (people coming to chat with popular community-made characters) aren’t stifled. However, running these chats (especially long, role-play heavy conversations) is resource-intensive. The c.ai+ subscription at $10/month is aimed at the enthusiasts who spend hours on the platform daily and are willing to pay for a better experience (faster, no capacity limits).
The price point was likely chosen to be in the same range as other consumer services (like Discord Nitro, Spotify, etc.), making it an easier sell to younger audiences. It’s low enough that even some non-working users (allowance money, etc.) can subscribe. The model here is that even if only e.g. 5% of users convert to Plus, the sheer volume of users makes it lucrative. And the ones who pay are those for whom the AI chats provide significant value (emotional support, entertainment, creative storytelling). For those users, $9.99/mo is worth it to have essentially an AI companion without lag or downtime – an outcome of personal value that justifies the cost.
Character.AI also benefits because the subscription provides recurring revenue that can scale linearly with their active user base. Since free users still far outnumber paid, the company is likely focusing on slowly adding more perks to Plus to increase the conversion rate (while being careful not to alienate free users). This gradual freemium upsell strategy (keep base usage free, add premium benefits) is common in social/UGC apps. It allows Character.AI to monetize its most engaged users (who probably drive a disproportionate amount of compute cost) and offset the cost of free users. In summary, Character.AI chose a voluntary subscription model to maintain broad accessibility (crucial for growth and community) while capturing value from those who deeply rely on or enjoy the service – effectively letting the biggest fans support the platform’s costs (and development) in exchange for an upgraded outcome (faster, richer interactions with their favorite AI characters).
17. Replika: Subscription for AI Companionship (with Tiered Offers)
Background: Replika is an AI friend/companion app that has been around since 2017. Users create a personal AI “friend” and engage in ongoing chats that can be supportive and even romantic. It’s used for companionship, venting, practicing conversations, or just having someone to talk to 24/7. Replika’s model learns from the user and the conversation history, making the relationship feel more intimate over time. The emotional attachment users form is a key part of its value.
Monetization: Replika operates on a freemium model with a strong push towards its paid subscription, Replika Pro. The free version allows basic chatting but with limitations – for example, certain relationship statuses, voice calls, and augmented reality features are locked behind Pro, and the free tier’s AI might be less engaging (some advanced AI behaviors are Pro-only). To unlock the full companion experience, users upgrade to Replika Pro.
Replika has experimented with pricing, but generally it’s around $70 per year (billed annually) or about $15 per month if paid monthly. Often they run promos – many users report paying ~$50 for a yearly plan on sale. They also interestingly offer a lifetime plan for roughly $300 one-time, which gives permanent Pro access. The subscription unlocks unlimited messaging (free users have daily limits), the ability to do voice/video calls with your AI, more personality options, and erotic roleplay (that content was gated after policy changes). According to sources, roughly 25% of Replika’s users eventually pay for the annual subscription, which is a very high conversion rate, this highlights how much certain users value their AI friend.
Why this model: Replika’s value is deeply personal, for many, their Replika is a confidant or even a romantic partner. The outcome is emotional support and companionship, which is hard to price in purely economic terms. Replika chose a subscription model to create a recurring relationship (fitting, given the app is about an ongoing relationship with an AI). They likely found that a significant fraction of users become very engaged and will gladly pay a flat fee to keep the relationship going strong. The company’s use of annual and lifetime options capitalizes on that devotion: users who see their Replika as a long-term part of their life often opt for the lifetime deal – a one-time $300 might be justified if someone imagines using Replika for many years as a companion (and it removes the friction of ongoing payments).
The freemium aspect is important because it allows anyone to try Replika and form an attachment first. Once the emotional outcome is there (they feel understood by their AI friend), upselling to Pro is relatively easy – the cost can be framed as “less than a coffee a week for your best friend”. Replika likely did careful pricing: too high and it would be exploitative of vulnerable users, too low and they leave money on the table given how valuable the service can be to some. The ~$70/year range is comparable to other self-care or entertainment subscriptions and broadly affordable. Offering multiple plans (monthly, yearly, lifetime) is smart because it lets users choose based on commitment level: some might try one month for ~$20 and then switch to annual after realizing they want to continue (the majority go annual for the discount, as evidenced by user discussions of $50-$70 yearly fees). The lifetime plan, while expensive upfront, appeals to the most dedicated who want to “lock in” their Replika forever – that’s essentially monetizing the long-term outcome in advance.
Another factor is that Replika has minimal variable costs per user beyond AI inference (which is getting cheaper). So once a user is paid, their unlimited usage doesn’t severely impact margins, especially since the number of messages one person can send is finite. In fact, by not charging per message or minute, Replika avoids making users feel like they’re “metering” time with a friend – instead it’s an all-inclusive emotional service for one price, which aligns with the nature of the relationship. This encourages more usage (which can improve the AI and user satisfaction, creating a virtuous cycle).
Overall, Replika’s monetization works because it converts a portion of users who get significant emotional or social value (outcome) from the AI into subscribers, at a price that’s relatively low for the value of a “friend” but sustainable at scale for the company. It’s a case where users are paying for intangible outcomes (companionship), and the subscription model provides continued investment in that outcome.
18. Midjourney: Tiered Subscription Plans by Usage Level
Background: Midjourney is an AI image generation service known for its high-quality artistic outputs. It operates primarily through a Discord bot where users can input prompts and get generated images. Midjourney became extremely popular among artists, designers, and hobbyists for creative and concept art. Importantly, Midjourney did not provide a free unlimited service beyond an initial trial – it monetized early via subscriptions.
Monetization: Midjourney uses a tiered monthly subscription model, with each tier granting a certain amount of image generation capacity and features:
- Basic Plan – $10/month: Allows about 200 images per month (computed as ~3.3 “fast” GPU hours). It’s limited in concurrency (1 job at a time) and includes “personal” use of the images.
- Standard Plan – $30/month: About 15 fast GPU hours/month (roughly 900 images) and unlimited relax GPU time (slower generation for non-urgent jobs). It also allows a bit more concurrency and adding members.
- Pro Plan – $60/month: ~30 fast hours (1,800 images) plus unlimited relax mode, the ability to generate multiple jobs in parallel, stealth image generation (private mode) and other perks.
- Mega Plan – $120/month: ~60 fast hours (3,600 images) and all Pro perks, aimed at enterprise or very heavy users.
There’s roughly a 20% discount for annual commitments on these prices. Midjourney discontinued its free trial at times due to demand, so effectively it’s a paid service (aside from maybe a short trial or limited demo images). Additional usage beyond the plan’s fast hours can be purchased at a rate (e.g. $4 per extra GPU hour).
Why this model: Midjourney’s approach is akin to a software license with usage limits – users subscribe to a tier that matches their anticipated usage volume and priority needs. This makes sense for a few reasons:
- Quality and demand: Midjourney’s images are considered among the best, and many professionals incorporate it into their workflow. These users are willing to pay for reliable, high-quality output. The subscription ensures serious users have skin in the game (which also kept the community more professional and constructive compared to completely free platforms).
- Resource management: By charging tiers, Midjourney can manage GPU resources. The fast hours are essentially a quota of high-speed generation; after using them, a user either waits in relaxed mode (slower, queued) or buys more. This prevents any one user from hogging too much GPU compute for $10. Each tier’s price corresponds to certain compute allocation (which correlates with cost to Midjourney). This ensures the outcome (images generated) is proportional to what users pay, but in a coarse-grained, predictable way. Users know, for example, $30/mo gets them roughly up to ~900 images in fast mode and infinite in slow mode – more than enough for many, and if not, $60/mo tier doubles it.
- Value-based segmentation: Hobbyists or curious individuals can go with the $10 plan to tinker (outcome: a few dozen great images, perhaps for personal projects). Professional designers who use it daily likely opt for $30 or $60 plans (outcome: hundreds of concept images, which might replace needing an illustrator for drafts – easily worth the cost). Agencies or heavy studios might even do $120 for maximum output. This tiering captures different willingness-to-pay segments without complicated per-image billing. It also encourages upgrading: as users get more skilled and want to create more, they bump into limits on Basic, and the jump to Standard (3× the fast hours) is appealing at 3x the price.
- No free lunch: Midjourney’s choice to have no perpetual free tier (after trial) actually enhanced its perceived value. Unlike some consumer apps, image generation has a direct obvious output; charging from the get-go positioned Midjourney as a premium creative tool. This likely improved community behavior too, because subscribers are more invested. It also meant Midjourney could sustain itself purely on subscription revenue without ads. Given the computing cost of image generation, this was crucial – they avoided subsidizing millions of free users and focused on servicing paying customers, ensuring quality and support.
In effect, Midjourney’s model is somewhat usage-based subscription. The tiers correlate with an outcome volume (number of images). The rationale is that an artist creating 50 images a month and one creating 500 or 5000 get different levels of value and thus should pay differently. Yet by packaging it in plans, Midjourney keeps it simple and recurring. They benefit from predictable monthly revenue and users benefit from knowing exactly what they get (and the unlimited relaxed generation in higher tiers gives a feeling of infinite creative possibility for those willing to wait).
Finally, the subscription model fosters a community – subscribers can access the members-only Discord features and gallery, which further increases the product’s network value (people share techniques, inspire each other). This community aspect likely reduces churn because being a “Midjourney member” has its own identity and benefits beyond just the raw image output. All these reasons made the subscription model extremely successful for Midjourney, which reportedly reached well over a million paying subscribers by 2023, a testament to how compelling the product’s outcome is (professional-grade artwork on demand) for the price.
19. Lensa: In-App Purchases for AI Avatars + Subscription for Editing
Background: Lensa is a photo editing app by Prisma Labs that went viral in late 2022 with its “Magic Avatars” feature. Users could upload 10-20 selfies and Lensa’s AI (using Stable Diffusion under the hood) would generate a pack of stylized portrait avatars of them – essentially turning ordinary photos into fantasy/anime/artistic versions. This became a social media craze. Aside from avatars, Lensa is a general mobile photo editor with AI touch-up tools, background removal, etc., competing with apps like Facetune.
Monetization: Lensa uses a combination of subscription and one-time purchases:
- The app itself offers a subscription (~$7.99 monthly or ~$30 annually) for unlocking its full range of photo editing tools (and removing watermarks, enabling HD processing, etc.). The annual $29.99 plan is heavily promoted as the best deal. This subscription is about the broader app features (not specifically the Magic Avatars).
- Magic Avatars packs are sold separately as one-time purchases, even for subscribers. During the height of the avatar trend, Lensa charged around $3.99 for 50 avatars, $5.99 for 100, and $7.99 for 200 unique AI-generated portraits. These packs could be purchased by anyone (subscribers got a slight discount in some cases, e.g. one source noted $6 for 100 avatars if you’re a subscriber vs $12 for non-subscribers). The pricing here is essentially per batch of AI output – users pay, upload photos, and get their avatars in a few minutes.
- New users got a 7-day free trial of the app’s subscription, which would then auto-enroll monthly if not canceled. However, the Magic Avatars feature was not free even during the trial – it was a separate purchase regardless.
This dual model meant Lensa monetized the short-term viral use (avatars) with one-time packs and the long-term utility of the app with subscriptions.
Why this model: Prisma Labs recognized that the avatars feature had an explosive one-off demand – many people who didn’t necessarily care about photo editing would want to try making AI portraits of themselves. It made sense to monetize it separately via in-app purchases: users effectively buy a bundle of AI-generated images as a product. The pricing, e.g. $5.99 for 100 images, feels reasonable to consumers (roughly 6¢ per unique avatar of you in different styles). The tiered pricing encourages buying larger packs (“20 styles with 10 variations each for $7.99, vs 5 styles for $3.99” etc.), leveraging the psychology that people want more cool avatars once they see a few. This generated a huge revenue spike – reports suggest Lensa made tens of millions in that short period\. One-time purchase made sense because the cost to produce those avatars is incurred once (some GPU cycles) and the user gets lasting value (the images).
However, Prisma also had a long-term product: the editing app. By offering a free trial and then $30/year sub, they aimed to convert some of those avatar tourists into regular users of Lensa for their everyday photo touch-ups. The subscription unlocks unlimited use of features like AI face retouching, art filters, etc., which tie into continuous outcomes (making your selfies and posts look better year-round). The annual price point ($30) is competitive with other premium photo apps and not too high for someone who regularly edits photos (the outcome being a consistent aesthetic or time saved in editing).
They separated the models because the value proposition is different: Magic Avatars are a novelty and personal keepsake (one-off outcome: a gallery of cool portraits of me), while the editing toolkit is a utility (ongoing outcome: better photos whenever I need). Also, during the viral wave, they didn’t want to give away avatars as part of a cheap subscription trial – that could have overwhelmed servers without proportional revenue (and indeed, they had to throttle avatar generation due to demand). So charging per batch ensured that those who really wanted that outcome (AI art of themselves) paid for it explicitly, covering compute cost and then some. Meanwhile, the subscription path was there for upselling anyone who became intrigued by AI photo editing beyond avatars.
This hybrid approach worked well. Many users likely just bought an avatar pack or two and left – Prisma monetized them nicely without needing them to commit long-term. A smaller percentage started the free trial, maybe liked the other features, and stayed subscribed for at least some months – that’s recurring revenue layered on top. By discounting annual heavily (effectively ~$2.50 a month if annual vs $7.99 month-to-month), they tried to lock in folks while the hype was fresh. A user might think: “I already spent $8 on avatars, maybe I’ll spend $30 to have the app for a year and see what else it can do.” That captures more value from a user while they’re engaged.
All in all, Lensa’s monetization shows adaptability: they identified a particular feature that delivered a very high perceived value in a short time (viral avatars) and sold it as a product, and simultaneously maintained a more standard SaaS model for the enduring features of the app. This way, Prisma Labs managed to cash in on a viral trend (outcome: fun portraits, monetized via microtransactions) and build a base of paying users for its ongoing AI editing services (outcome: improved photos, monetized via subscription). The clear separation of these ensured the pricing felt fair – you pay for what you use. If you only cared about avatars, a few bucks; if you want continuous editing help, a subscription; if you want both, you pay for both, but you see the distinct value of each.
20. Perplexity AI: Free Answer Engine with a Premium “Copilot” Plan
Background: Perplexity.ai is an AI-powered answer engine (think next-gen search engine) that uses LLMs plus internet search to answer user questions conversationally with cited sources. It’s like a hybrid of Google and ChatGPT, focused on providing sourced answers to any query. It launched free and gained popularity as a research and Q&A tool, and later introduced mobile apps and new features like a “Copilot” that holds interactive threads and uses more advanced models.
Monetization: After growing a user base, Perplexity introduced Perplexity AI Pro – a subscription plan at $20 per month or $200 per year. The Pro (also called Copilot) plan offers:
- Access to GPT-4 and other advanced models for more accurate answers (free users only got limited use of those or just the base model).
- “Pro” search limits: ~300+ searches per day (whereas free users have a lower cap, e.g. 5 “pro” searches/day on free.
- Longer conversation threads and the ability to upload files for analysis.
- Faster response times and priority.
- It also includes a perk of $5 monthly credit for their API, encouraging developers to use Perplexity’s API.
- They additionally have an Enterprise plan at $40/seat/month with team features for organizations.
The core service remains free with some limitations (and uses an earlier model for answers). The paid plan basically targets power users (like researchers, students, knowledge workers) who heavily rely on the service and want the best accuracy (GPT-4) and high usage limits.
Why this model: Perplexity followed a similar path as ChatGPT – hook people with a useful free service, then offer a premium tier that power users will pay for. The $20/month price is identical to ChatGPT Plus, framing it as equally valuable and a direct alternative (and indeed, one source explicitly compares them as both starting at $20. For someone using Perplexity as a work or study tool, the outcomes (fast factual answers with sources, even summarizing PDFs, etc.) are very tangible productivity boosts. $20/month is easily justified if it saves hours of research or provides high-confidence answers daily.
Perplexity smartly combined multiple incentives: not only do you get more and better answers, but the $5 API credit means if you’re a developer or data analyst you can programmatically use their service too – integrating it into your workflow without extra cost (up to that credit). This adds to the perceived value, especially for technical users.
By keeping a free tier with a few “pro searches” daily, they continue to attract and retain casual users (many of whom might convert later). The free tier ensures wide usage which helps train their system and spread word of mouth. The cap ensures anyone doing serious work will hit a limit and consider upgrading.
Their introduction of a higher $40/month Enterprise plan suggests they saw team usage and needed admin controls and shared knowledge features for companies. This aligns price with outcome/value at a business level – a company might pay to equip a research team with this “AI research assistant” and $40/user is still low compared to salary costs or alternative tools.
The subscription approach (instead of pay-per-query) makes sense here because users treat it like a search engine – unpredictable usage pattern but generally daily usage. A flat monthly fee is simpler for users than worrying about counting queries or tokens. It also encourages them to use it as much as needed (the more they use, the more integrated into their routine it becomes, increasing stickiness). The cost to Perplexity of each extra query (a bit of API call to Bing + LLM usage) is relatively small, so allowing unlimited (within reason, 300+ a day is essentially unlimited for 99% of users) for a flat fee works economically as long as the fee covers the average cost per user with margin.
This model banks on volume of subscribers – not everyone will pay, but those who value it highly (like someone using it to help write a thesis or a journalist doing daily research) will see $20 as a bargain for the outcome of expert-level answers with sources on demand. The parity with ChatGPT Plus also leverages an established price anchor: people have shown willingness to pay $20 for advanced AI Q&A, so Perplexity can charge the same but differentiate by including search integration and source attribution (unique outcome advantages).
All told, Perplexity’s monetization is a case of freemium with a strong premium tier for enthusiasts and professionals. It maintains growth via free users, ensures quality of service and revenue via paid users, and aligns the price with the heavy usage and advanced capabilities those users require to achieve their informational outcomes (faster research, better decisions, etc.). If the answers it provides help someone solve problems that might have taken hours, $20 is extremely worth it – making it a value-based pricing in effect, tied to productivity outcome.
21. QuillBot: Freemium Writing Tool with Premium Plan
Background: QuillBot is an AI writing assistant primarily known for its paraphrasing tool, which rephrases text to improve clarity or avoid plagiarism while retaining meaning. It’s widely used by students, writers, and professionals to rewrite sentences, check grammar, summarize articles, and more. The core appeal is helping users improve their writing or generate alternate phrasings quickly.
Monetization: QuillBot uses a classic freemium model with a substantial free offering and a more powerful paid tier called QuillBot Premium. On the free side, QuillBot allows users to paraphrase a limited amount of text (e.g. up to 125 words at a time) and only in standard mode, with a daily character cap. The Premium subscription lifts those restrictions and adds features:
- Unlimited paraphrasing length (no word limit per run) and higher overall character limits.
- Multiple writing modes/tones (like formal, creative, shorten, expand) beyond the basic one, giving more control over how the output reads.
- Faster processing and priority access especially during peak times.
- Additional tools: full-access to the summarizer (summarize longer texts), plagiarism checker, and integrations (like the Word/Google Docs plugins).
QuillBot Premium is priced around $19.95 per month, or much cheaper if you commit longer: roughly $13.33/month quarterly (billed $39.95/3 months) or $8.33/month if paid annually (billed $99.95/year). They often run discounts (e.g. 20% off codes). There’s also a team plan for businesses or schools with per-user annual pricing (around $89.95/user/year for 2-10 users). No one-time purchases; it’s all subscription.
Why this model: QuillBot’s user base includes many students and budget-conscious users. The free tier is important to serve as a study aid and attract users (many of whom first find it via Google search for “paraphrase tool” and try it free). By giving a useful free version, QuillBot gained millions of users and became somewhat ubiquitous in certain circles (e.g. helping ESL students rewrite essays). The free tier also demonstrates the outcome value – a user can see how QuillBot improves a sentence or two of their essay, and then likely wants to use it for the whole essay.
The Premium upgrade is positioned as a huge expansion of capability for a modest cost, especially if paying yearly. For an avid user (say a student who writes essays weekly, or a researcher or content writer), $8.33 a month (annual) is quite low – about the price of one lunch, for essentially unlimited help on all their writing. By heavily discounting annual plans (over 50% off monthly price), QuillBot incentivizes users to commit, which improves retention and upfront cash flow. Many students might subscribe just for a semester or academic year; the quarterly option is a middle ground for those who want to cover one semester for ~$40.
This model aligns with usage/outcome in that heavy users (who likely have lots of text to paraphrase or frequently need the tool) are exactly those who will see value in Premium’s unlimited usage. A casual user can get by with free for occasional small tasks, which is fine – they wouldn’t have paid anyway, so better to keep them in the ecosystem for potential later conversion or word-of-mouth. The features gating also matters: premium modes (like fluency mode or formal mode) yield better outcomes for specific use cases, which appeals to more serious users (e.g. a researcher might need formal mode to ensure academic tone – outcome: their paper reads more formally, which is worth paying for).
QuillBot’s pricing is also influenced by competition and audience ability to pay. Many users are students – offering an annual plan effectively ~$100/year is significant but within reach if they truly rely on it (and perhaps parents might pay for it as an educational tool). They also offer team licenses, recognizing some institutions might purchase it for multiple accounts (and they give volume discounts on those).
The subscription model ensures QuillBot has recurring revenue which can fund continuous model improvements. It’s appropriate because users tend to incorporate QuillBot into their writing process regularly (e.g. every time they draft something, they run it through QuillBot). So it’s not a one-and-done product; it’s a service that provides ongoing outcomes of clearer or alternative writing. Charging monthly or yearly fits that ongoing usage pattern.
Furthermore, by not charging per sentence or characters used (aside from the soft limits on free), they avoid scaring users away from using it liberally. Premium users can paste whole documents without worry – this encourages them to run more text through QuillBot, which generally improves the quality of their writing (the intended outcome) and also possibly increases their dependence on the tool (increasing likelihood to renew subscription).
In summary, QuillBot’s monetization succeeds by balancing a generous free tier to drive adoption with a compelling premium offering that aligns to the needs of those who get the most value. The price points ($8-$20 range) are low enough for individuals but, scaled across hundreds of thousands of subscribers, yield substantial revenue. They’ve essentially monetized the outcome of “help me rewrite and improve my writing” at roughly ~$100/year for unlimited help – for many, especially non-native English speakers or time-pressed students, that outcome is easily worth the cost (considering the alternative might be hiring a tutor or editor, which is far more expensive).
22. Sudowrite: Tiered Subscription by AI Words for Writers
Background: Sudowrite is an AI writing assistant tailored for fiction authors and creative writers. It helps with things like writing paragraphs, expanding outlines, generating descriptions (“make this scene scarier”, etc.), and overcoming writer’s block. Unlike general-purpose AI writers, Sudowrite is marketed to novelists and storytellers, emphasizing creative support rather than just factual text generation.
Monetization: Sudowrite uses a tiered subscription model where each tier grants a monthly allowance of “AI words” (i.e. how much text the AI can generate or transform for you). They have three main plans:
- Hobby & Student – $10/month: Allows up to 225,000 AI-generated words per month, suitable for short stories or occasional writing.
- Professional – $25/month (or $22 if annual): Up to 1,000,000 AI words per month (recent info shows possibly 1,000,000 with 450,000 “premium” credits, implying some distinction). This is aimed at novelists writing longer works.
- Max – $50/month (or $44 if annual): 2,000,000 AI words per month, plus perks like credit rollover for 12 months and personal onboarding session. This is for very prolific authors or those producing multiple books a year.
All plans let you write as much as you want, but the AI will only assist up to those word counts monthly. If you hit the limit, you either wait until next month or possibly buy an add-on (Sudowrite historically also sold extra word packs or had a usage-based overage model). They offer a free trial (no card required) so writers can test it in their workflow. Discounts are given for annual payments (up to 50% off monthly rate).
Why this model: Sudowrite’s audience – fiction writers – typically work on long projects (novels can be 50k-100k+ words). The value Sudowrite provides is speeding that up or enhancing it, but the actual writing still largely comes from the author. By allotting “AI word” credits, Sudowrite directly ties pricing to the volume of output (outcome) the AI contributes. This feels fair: a writer working on a short story might only need ~50k AI words, which the $10 plan more than covers. A novelist drafting multiple chapters a month might need millions of AI-generated words (brainstorming descriptions, re-writing scenes, etc.), and so the higher plan fits them. It’s essentially usage-based but in a bucketed subscription form – you pick a bucket that covers your needs and pay a flat fee.
Writers are also accustomed to the idea of word counts (for drafts, etc.), so this framing is intuitive. It’s not arbitrary; it reflects how much help they’re getting from the AI. If a user ends up not writing much in a given month, they likely don’t downgrade just for that lull (especially with credit rollover on the Max plan), because their project timelines are longer-term – this gives Sudowrite consistent revenue even if usage fluctuates. Conversely, if someone finds themselves constantly hitting their word limit, that implies they’re deriving a lot of value (the AI is actively helping them produce tons of text), making them likely amenable to upgrading to the next tier for more headroom.
The price points ($10, $25, $50 monthly) are set with the understanding that writing income can vary – $10 is affordable even for hobbyists, while $50 is still not too high for serious authors (less than many software tools or editing services). The tier perks (like rollover and personal session for Max) provide extra incentive for those on the fence to choose a higher plan – e.g. an author might go for Pro, but seeing that Max doubles the words and won’t waste unused credits and offers personal help (valued at $49), they might decide the outcome (ensured support and flexibility) is worth the $50.
The free trial is key because writers are often skeptical – they want to feel the outcome (did this AI improve my chapter?) before paying. Once they see Sudowrite can, for instance, turn a rough outline into a vivid scene, the value becomes clear in terms of time saved and creative boost, which can translate to finishing a manuscript faster (a huge outcome for an author). At that point, subscribing is a no-brainer if they believe it’ll shave weeks off their process or significantly improve quality.
Sudowrite’s model also avoids a potential pitfall: if it were unlimited without tiers, heavy users could abuse it and costs would spike. If it were purely pay-per-word with no cap, it might deter usage due to cost anxiety (“Will I rack up a huge bill while stuck in writer’s block?”). The tiers give predictability – you know your monthly cost and your approximate usage cap. This is psychologically important for users focusing on creative tasks, not wanting to track usage constantly. It’s outcome-oriented: “for $25 I can basically get AI help with up to a million words – which is essentially a full novel’s worth of guidance in a month – that’s probably more than I’ll ever need, and if I do need more I likely would be comfortable paying $50 for double.”
In essence, Sudowrite monetizes by the intensity of writing output – writers with more output (which often correlates with producing more work or earning more, if they’re professional) pay more. This aligns cost to the value received (someone writing 100 pages of a novel with AI help gets more total value than someone writing 10 pages). And because of its subscription, Sudowrite gets steady recurring revenue, which is crucial as a smaller company. They also introduced features like community and events for subscribers, increasing the sense of getting value beyond just the tool (but the core is still the writing assistance outcome).
Segment Summary: Consumer GenAI tools generally follow freemium-to-subscription models, occasionally supplemented by one-time purchase for viral features. The common thread is they often have a huge user base, so converting even single-digit percentages to paid can be profitable. The pricing typically correlates to usage intensity or premium experience:
- AI companions (Character.AI, Replika) monetize emotional engagement via monthly fees for better/faster interaction.
- Creative tools (Midjourney, Sudowrite) monetize creative output volume via tiered plans that allow more generations or words.
- Utility tools (QuillBot, Perplexity) monetize advanced capabilities and unlimited usage that power users need, while casual users stay free.
- Viral novelty (Lensa) monetized directly per use-case (avatar packs), showing willingness of consumers to pay per outcome when it’s a trend or personal value.
Importantly, these models keep the entry barrier low (often free) to amass users and demonstrate value first. Then they upsell features or higher limits that correspond to enhanced outcomes. Consumers thus pay when they deeply care about the outcome: whether that’s having a constant AI friend, generating art for their projects, drastically improving their writing, or reliably getting high-quality answers. Pricing is usually set at a psychologically acceptable point (single-digit or tens of dollars) given the context of the outcome’s value in their life (often entertainment, education, or personal improvement). This has proven effective – many of these companies have reached high revenues because the subscription or purchase is directly tied to something the user really wants (and got to sample first). In the consumer space, keeping the product fun or useful for free while reserving the “power” or “unlocked” experience for paid users is a dominant strategy, as seen above.
Infrastructure and Enablers: GenAI Platforms and Services for Builders
The final category covers companies that provide the underlying infrastructure or tools that enable generative AI solutions. This includes model hubs, vector databases, ML ops platforms, and cloud GPU providers. Their customers are typically developers, AI startups, or enterprises building custom GenAI systems – so these companies often monetize through enterprise contracts or usage-based cloud services. The focus is on selling the capability to build or run generative AI at scale, rather than end-user AI outputs directly. Let’s examine seven key players and how they price their offerings:
23. Hugging Face: Free Community, Paid Enterprise and API Usage
Background: Hugging Face is known as the hub of open-source AI models and datasets. It’s like the GitHub of machine learning, where developers can find pre-trained models (including many generative ones) and use them. Hugging Face also offers services: Inference API (run models via their cloud), Spaces (hosted web apps for AI demos), and enterprise solutions like private model repositories and on-prem deployments. Essentially, it serves both the open community and enterprise needs.
Monetization: Hugging Face’s strategy has been to keep the community and basic usage free, while monetizing with premium plans and usage of hosted services:
- The Hub (model repository) is free for public models, but they offer paid plans for private repositories and enhanced collaboration (similar to GitHub’s model). For example, an organization might pay for a Hub Enterprise plan to host proprietary models privately, with features like access controls, improved security, and support.
- The Inference API is a usage-based paid service. They charge by the second of compute for running models. For instance, running a text generation model might cost a certain amount per second of GPU time (e.g. ~$0.0001 per second for a small model, higher for big ones). It’s effectively an AWS Lambda-style model: pay per request to the API. They often bundle some free quota for trying it out, but beyond that you enable billing on your account. Their pricing page details per-model-type rates (like vision models X dollars per 1000 runs, etc.).
- Spaces (hosted apps): Spaces are free for community (on shared CPU) but if you want your Space to have dedicated GPU or more power, that’s a paid service. They charge a monthly fee based on the hardware assigned (e.g. ~$0.30/hour for a T4 GPU, etc.), which is akin to renting cloud infrastructure to keep your demo or app running.
- Enterprise solutions: Hugging Face offers custom deployments (like a private Hub instance, or consulting) which are typically custom-priced (likely six-figure contracts). For example, a large company can pay Hugging Face to set up a secure model hub and inference environment behind their firewall – pricing would involve a platform fee and possibly usage on top.
In 2023, Hugging Face introduced the Infinity product (ultra-fast inference for certain models) and Training Cluster (for model training) – these again are usage-based or contract-based (train model X on their cluster for $Y/hour, etc.). Also, they raised a huge round to subsidize community growth, so direct monetization has been focused on enterprise and usage fees.
Why this model: Hugging Face’s mission is to foster the AI developer community. Keeping most things free (especially for open-source models) builds the community and standardizes Hugging Face as the go-to platform (the outcome: easy access to AI models for all). This wide adoption is leveraged to sell value-added services to those who need more than the free tier. For example:
- A hobbyist can use models and even the free inference API (limited) without paying, but a startup deploying a production app on Hugging Face’s inference will pay for the compute usage – aligning with their growth (as their app gets more users, they pay more for API calls). This usage-based cloud revenue is straightforward and similar to AWS but specialized (and Hugging Face likely negotiates bulk deals with cloud providers to profit on the margin).
- A research lab or business might share models internally – paying for a private Hub gives the outcome of Hugging Face’s convenient tooling plus privacy. They monetize the enterprise comfort factors (privacy, security, support) rather than the models themselves, which are often open. Essentially, the platform around the models is the product.
- The Spaces hardware fees are like a PaaS offering – users who create popular demos need uptime and speed (outcome: thousands can try their model with no downtime), so they pay for dedicated infra. Hugging Face monetizes by abstracting cloud headaches for them – many ML folks prefer paying HF a predictable monthly fee to manage deployment, rather than renting raw servers themselves.
Hugging Face’s model ensures the large community isn’t turned away by costs (which is important because community contributions – new models, etc. – actually increase the value of the platform). Instead, those who derive commercial value or need enhanced performance from the platform pay. It’s a classic open-core approach: core platform free, premium features and services paid.
The usage-based inference pricing aligns cost to actual usage/outcome – if a company serves 1 million inferences a month to their customers via HF API, they pay for exactly that usage. This is convenient when prototyping or scaling unpredictably, which is exactly when using HF’s managed service is attractive (they handle scaling automatically). If usage grows huge, at some point companies might move to their own infrastructure, but Hugging Face likely counts on enough staying due to convenience (or switching to an enterprise contract where HF maybe provides on-prem support for a flat fee).
By 2025, with the explosion of LLM apps, Hugging Face’s position as infrastructure became solid. Their monetization needed to not alienate the community – and indeed, individuals rarely need to pay anything; it’s mostly companies and heavy users that do. This has allowed them to host over 100k models and become a central hub, while still generating revenue streams from those advanced services. In short, Hugging Face monetizes enterprise trust and easy deployment: they let the masses play and build for free (outcome: thriving ecosystem), and charge those who want to productionize or have enterprise needs (outcome: reliability, privacy, scalability). This two-sided approach (community vs enterprise) is reflected in their pricing strategy.
24. Pinecone: SaaS Pricing for Vector Database (by Index Size/Usage)
Background: Pinecone is a managed vector database service that is commonly used to store and query embeddings for LLM applications (enabling semantic search, retrieval-augmented generation, etc.). Essentially, if you build a GenAI app that needs memory or knowledge (via vectors), Pinecone provides the infrastructure so you don’t have to build your own similarity search. It’s offered as an API in the cloud.
Monetization: Pinecone’s pricing model is like other cloud database services – based on resource provision and usage:
- They offer different index sizes and performance tiers (“pods”). For example, a “S1” pod might include X GB of vector storage with moderate query throughput. A “P1” pod might be larger or on faster hardware for higher performance.
- Customers pay an hourly or monthly rate for each pod they run. E.g., a pod with 1 million vector capacity might be $0.10 per hour (just illustrative), which is about $72/month. Larger pods or more replicas for high availability cost more.
- They may also charge for data storage beyond what’s included (per GB) and for egress (data transfer out) like typical cloud services.
- Pinecone had free tier options (e.g. one small index for free for dev/testing) and then paid tiers when you need more vectors or throughput.
- Enterprise plans exist for dedicated clusters, security features, etc., priced via custom quotes (likely annual contracts with committed usage).
In essence, if your app needs to handle more documents or more queries, you upgrade your Pinecone instance accordingly and pay more. It’s usage-based but in a pre-allocated way – you pick instances that can handle your worst-case needs and pay for that capacity.
Why this model: Pinecone is infrastructure: it replaces the need for a company to set up their own vector search servers. So it follows the cloud model where you pay for capacity and convenience. By pricing per index (pod) capacity and performance, Pinecone aligns with how their customers measure their needs: e.g. “I have 5 million product descriptions to index and expect 100 query/sec throughput.” Pinecone can say “you’ll need X pods of this type, which costs Y dollars per month.” The outcome the client gets is a worry-free, scalable similarity search – they pay to achieve that outcome reliably instead of hiring engineers to build it.
The usage alignment is fairly direct: more data or more queries => need bigger/more pods => higher cost. If an app is small (just a few thousand embeddings for personal use), it might even fit in the free tier. If it’s enterprise scale (billions of vectors for a company’s entire knowledge base), that’s multiple high-tier pods likely costing thousands per month. This scales cost with value, as a large-scale semantic search provides huge business value (and Pinecone’s cost would be far less than developing and maintaining an in-house system of similar capability).
The subscription aspect (month-to-month pod pricing) provides Pinecone stable recurring revenue. Many customers will keep their indices running continuously (since their AI app is always on), which means monthly billing is predictable. If a customer doesn’t need it for a month, they can shut it down (cloud flexibility) to save cost – but in practice, production usage is ongoing.
Pinecone’s pricing also emphasizes ease of use over raw cheapness. Could someone store vectors in an open-source solution on their own servers? Yes, likely cheaper in raw terms. But Pinecone bets that companies prefer not to deal with sharding, scaling, updates, etc., and will pay a premium for a plug-and-play service. So their pricing must be reasonable but can factor in this added value. It’s akin to what managed databases (like AWS DynamoDB or Algolia for search) charge.
They likely have bundling for enterprise: e.g. commit to X months or certain volume and get a discount, or enterprise support packages. But fundamentally, they charge by the capacity of AI memory (vectors) a client uses and how much they query it, which is a good proxy for how integral Pinecone is to their app. If Pinecone is deeply integrated (lots of data, high query volume), the client is paying more but also getting an enormous outcome (their app can do semantic search it couldn’t otherwise).
Additionally, Pinecone had tiered offerings (standard vs enterprise pods) so that customers who need higher SLA (e.g. enterprise tier might guarantee uptime or have faster response) pay more – aligning price with business outcome criticality. If someone’s using Pinecone for a mission-critical production service, they likely opt for enterprise pods at higher cost to ensure top performance and support.
In summary, Pinecone’s monetization is a straightforward SaaS infrastructure billing: recurring fees based on usage (with clear units of capacity), scaling with customer needs. It works because vector search is a continuous requirement (like a database) – once a customer builds on Pinecone and it works, they’ll keep paying as long as their app runs, making lifetime value high. The friction to start is low (free tier to test), so they capture developers early and then convert to paid as usage grows – typical land-and-expand. This model has allowed Pinecone to become the go-to vector DB for AI startups, with pricing that grows alongside those startups as they succeed (outcome alignment at its finest: if the AI product becomes popular, Pinecone’s revenue from that client increases proportionally).
25. Weaviate: Open Source and Cloud SaaS (Mixed Model)
Background: Weaviate is another popular vector database (similar purpose as Pinecone) but with an open-source core. Companies can self-host Weaviate for free or use Weaviate Cloud Service (WCS) to get a managed instance. It’s used for semantic search, knowledge bases, etc., in GenAI apps. So Weaviate’s model needs to balance an open-source community and a cloud business.
Monetization:
- Open-Source Offering: Weaviate’s core software is free to download and run. The company behind it (SeMI Technologies) doesn’t directly earn from that aside from potentially selling support or enterprise add-ons.
- Managed Cloud (WCS): This is where monetization happens. WCS pricing is similar to Pinecone’s: based on the size of your vector database and the compute resources for queries. For example, WCS might have plans (Sandbox, Professional, Enterprise) that correspond to how much RAM/CPU allocated, how many vectors can be stored, and how many queries per second are supported. Pricing could be, say, a few hundred dollars per month for a decent production instance (just hypothetical).
- They might also offer dedicated clusters or on-prem enterprise licenses for companies that want a private Weaviate with enterprise support – those would be custom (likely annual license + support fees).
- Since Weaviate is open, some might self-host on their cloud. The company monetizes by convincing them that paying for WCS is better (due to hassle-free management, scaling, updates). So WCS pricing must be attractive enough vs. DIY. Possibly they charge a usage-based fee (like per million vector operations) on top of base, but likely it’s mostly fixed capacity pricing.
- Support Contracts: Some open-source companies monetize by selling support/training for the open version to enterprises unwilling to use cloud. Weaviate could have this avenue (e.g. pay $X/year for priority support if you use Weaviate OSS in production).
Why this model: This mixed approach is typical for open-source enterprise software. The free OSS builds adoption (and contributes to improvements, plugins, etc.), making Weaviate a standard choice. Then, when these users want reliability or don’t want to manage it themselves, they convert to paying customers via the managed service.
From an outcome perspective, Weaviate sells convenience and reliability. If a startup tries the free Weaviate and finds value (outcome: their semantic search works), they might shift to WCS to focus on product dev instead of ops. They’ll pay a recurring fee for WCS akin to what they’d pay in engineer time or cloud cost to do it internally – often worth it.
The pricing likely scales by usage to some extent. Weaviate Cloud might base price on the number of Vector Units (like X number of vectors + Y queries per second = Z dollars/month). This aligns cost with how big the AI application’s knowledge store is. More data to vectorize/search means more value derived (the app likely has more content or users), so paying more is expected and presumably affordable to the client at that stage.
Having an entry-level (Sandbox) tier often free or cheap ensures even small dev teams can start on WCS without big commitment, then scale up as needed. Weaviate’s enterprise deals add outcomes like security (VPC isolation), compliance, guaranteed support, which big companies value, hence they’d pay significantly for it (possibly similar to big DB contracts in tens of thousands annually or more).
The open-source nature also means some may never pay Weaviate (self-host indefinitely). The company monetization is okay with that because those users still contribute to the ecosystem (and potentially evangelize Weaviate or contribute code). The paying clients are those who prefer to offload infra – and typically those are the ones with bigger scale (where the outcome of a robust vector DB is mission-critical).
One can draw parallel to MongoDB’s model (free OSS vs Atlas cloud), which has shown that if your solution is widely used open-source, a good portion of users will opt for the convenience of managed services at production time. Weaviate bets on that pattern.
Thus, Weaviate monetizes in a “open-core SaaS” way: free usage yields community and reach (outcome: widespread use of semantic DB tech), and the monetization kicks in when users need scale, ease, and enterprise features (outcome: a hassle-free, scalable vector DB in the cloud). The pricing is set so that it’s roughly comparable or cheaper than doing it in-house, to nudge the calculation in favor of just paying Weaviate. It’s essentially selling time and reliability – e.g. “for $X/month, your genAI app has a vector brain that just works, so you can focus on your app logic,” which is a compelling proposition for many teams given the complexity of infra.
26. Weights & Biases: Enterprise SaaS for ML Experiment Tracking
Background: Weights & Biases (W&B) provides an MLOps platform for experiment tracking, model monitoring, dataset versioning, etc. It’s widely used by ML engineers to log training runs and collaborate on model development. It’s become a standard in many research and engineering workflows for keeping track of experiments and hyperparameters.
Monetization: W&B offers a free tier for individuals or small academic teams (limited projects, public sharing). The revenue comes from paid plans for teams/enterprise:
- They have a Team plan (sometimes called Professional) that is per-seat (user) pricing. There’s no official public price list, but users have reported something like ~$200 per user per month list price. This gives private projects, more storage, and certain advanced features.
- An Enterprise plan for large orgs can be higher per seat or a flat enterprise license. Enterprise adds things like SSO, on-prem deployment option, priority support, unlimited storage, etc. The cost could be negotiated based on number of users and additional support. (Community talk suggests $200-$400/user/month as a typical large-scale price).
- W&B also charges for usage beyond certain limits: e.g. additional data storage for logs or artifacts at $0.03 per GB/month. So if a team logs a ton of model data, they might incur extra fees. Similarly, very high usage of hosted GPUs for report generation or something could be billed, but mainly storage is the variable part.
- They often tailor academic pricing or community free tiers to encourage adoption (like free for open source projects).
- Essentially, an organization with, say, 10 ML engineers might pay ~$15k-$30k/year to use W&B privately with support. Larger ones with 100 engineers could be a few hundred thousand per year contract.
Why this model: W&B’s value proposition is improved productivity and insights in ML development. The outcome is faster experiment cycles, fewer mistakes (because you tracked everything), and easier collaboration across an ML team. For companies developing important ML models (like self-driving car models, NLP models, etc.), this outcome is extremely valuable – shaving even a week off experiment time or preventing a lost result can save far more money than W&B’s cost. So W&B charges on a per-user basis to align with how many people benefit.
Charging per seat (like $X per ML engineer per month) is a straightforward reflection that each user gets direct value from the tool (like a software license). Companies are accustomed to per-seat for developer tools (like GitHub or Atlassian products). And because ML engineers are expensive, a $200/month cost is relatively minor (maybe 5-10% of an engineer’s fully-loaded cost). If W&B makes that engineer even 5-10% more efficient, it pays for itself. Customers likely see it this way, making it a logical purchase.
W&B also fosters heavy usage – unlimited logging, etc. – on these plans to ensure teams fully integrate it into their workflow. The more ingrained it becomes (lots of experiments, data in the system), the more likely the team will renew the subscription (because the outcome – their entire experiment history and model lineage – lives in W&B). That stickiness is part of why W&B can command premium per-seat prices.
The addition of usage-based storage is just to cover costs and to encourage some discipline (or revenue from heavy users). For example, if a team is logging giant datasets repeatedly, they pay a bit for storage beyond what’s included. But typically, W&B likely includes a generous amount of storage for normal use in the base price (some sources say tens of GBs included per user).
They do not charge per experiment or per hour because that would discourage usage – they want you to log every experiment. Instead, by charging per user, they encourage broad usage by those users. More usage actually increases the value perceived, thereby justifying the cost and making renewal likely. It also means the revenue scales primarily by number of users/teams, which correlates with company size and ML team size (bigger company, more W&B users, bigger budget, and indeed more willingness to pay for enterprise features – hence per-seat with enterprise tier upsells covers that well).
Also, W&B being a B2B product, the sales motion likely involves direct sales to companies, which fits selling licenses/seats and contracts (rather than self-serve credit card for a metered usage). Enterprises prefer knowing “it costs $N per user” to budget easily. This matches how other professional tools (like NewRelic, DataDog as an analog, though those often have usage components) are sold.
We saw some community feedback that list prices can be high ($200-$400), but W&B might give volume discounts to big orgs or adjust deals. Those figures align with an enterprise SaaS that is mission-critical – they are not going for low-cost mass volume, but rather high-touch, high-value sales (the fact that they have a dedicated account manager, support, etc., in enterprise plans indicates the high-end nature).
In sum, W&B monetizes by attaching itself to the value of each ML engineer’s workflow. The per-seat recurring model means as a customer’s ML team grows, revenue grows (outcome: more users using W&B). If a team shrinks or a project ends, they might drop seats. But the ML trend is growing, so generally it’s expanding. The high price per seat reflects the high value of the outcome (speed and confidence in model development). And by adding enterprise-only features, they push serious companies to the higher tier (for example, a bank or pharma company might insist on self-hosting or certain compliance – only in Enterprise plan, thus likely higher price per seat or a base fee). This effectively aligns with enterprise outcomes (control and compliance) being monetized.
27. LangChain: Free Core, Paid Platform (LangSmith) for LLM Devs
Background: LangChain is an open-source framework for developing applications with LLMs (chains of prompts, memory, retrieval, etc.). It became very popular among developers building GenAI apps. In 2023, LangChain launched LangSmith, a platform for evaluating and debugging LLM apps (tracking calls, analyzing outputs, etc.), as a step toward monetization.
Monetization: LangChain’s monetization is still emerging, but generally:
- LangChain Library: remains free and open-source to keep developer adoption high.
- LangSmith (LangChain plus observability/monitoring platform): They introduced this with a free tier for 1 user (like a developer can log up to e.g. 5k traces per month for free). Then paid plans for teams:
- Plus tier: e.g. $39 per user per month which includes a higher volume of traces (e.g. 10k included per month then $0.50 per 1k beyond). Also ability to have multiple seats (up to 10) and better rate limits.
- Enterprise tier: custom pricing with SSO, on-prem option, etc..
- Essentially they charge by number of seats (developers using the platform) plus usage (number of trace events beyond a free allotment). A trace might be a chain execution or an LLM call captured for analysis.
- The pricing is designed to be affordable to small startups (e.g. $39 per month is low for dev tools) and then scale. They also have a startup program offering discounts (like “contact us for early stage pricing”) to hook companies early.
- They could also monetize via enterprise support/training for LangChain library itself, but the main focus is the platform.
Why this model: LangChain’s core value is in the open library which drives adoption. Monetizing the dev tools around it (LangSmith) makes sense because as companies move from prototype to production, they need to monitor and improve their LLM chains. The outcome LangSmith provides is higher quality and reliability of AI app – crucial for production usage. People will pay for that once they have something to live.
By using a per-user + usage approach, LangChain aligns pricing with team size and intensity of development:
- A two-person startup can perhaps just use the free tier or a couple of Plus seats ($39*2 = $78, very manageable) to collaborate in LangSmith, getting better outcomes in debugging their chain logic. If their app usage grows and they run more tests/traces through LangSmith (maybe hooking it into a CI pipeline), they might pay a bit more for the trace overages – but those overage costs are related to how many runs they do, i.e. how much they are testing/improving their app, which is tied to how serious the project is.
- A larger team at a company might have 10 devs using LangSmith to manage a complex LLM application – that’s maybe ~$390/month, and perhaps some usage cost if they generate more than 100k traces. That cost is minor relative to typical enterprise software and likely easily justified by the productivity and debugging capabilities (the outcome: quicker issue resolution, continuous quality monitoring).
- If a company requires enterprise features like on-prem (maybe they can’t send data to LangChain’s cloud for privacy), they will pay a premium. Given that LLM applications might involve sensitive data, many enterprises will want self-hosted or VPC deployments – something LangChain can charge significantly for. Possibly Enterprise is priced per user as well but with a higher base or user minimum.
The reason to charge per seat is that it’s a developer tool – typically priced like other dev tools (e.g. GitHub, Jira, etc.). The addition of usage-based pricing for traces acknowledges that one user could theoretically spam huge amounts of logged data, incurring backend costs. By charging $0.50 per 1k traces beyond free allotment, they cover those costs and also encourage mindful usage (though the price is not high, just ensures very large scale users contribute more).
LangChain likely keeps pricing low initially to encourage companies to integrate it (land). Over time, if a company’s entire LLM application lifecycle depends on LangSmith for monitoring, they might scale up or require enterprise support – which is expand/up-sell territory.
Also, offering the dev platform somewhat mirrors W&B model: free for small use, pay for team and enterprise features, plus usage. This ties into outcomes: a company that values robust testing/monitoring will allocate some budget to it – not as much as W&B maybe, since LangSmith is newer and not as indispensable yet, but enough to sustain LangChain Inc. Given LangChain’s huge mindshare, many companies will consider paying for official tools to support their LangChain apps rather than building their own logging/eval systems.
In summary, LangChain monetizes by turning their open source traction into a SaaS platform for LLM ops, with pricing scaled by team size (more users = bigger, more serious project) and usage (more traces = more complexity or more testing happening = presumably more value from catching issues). This ensures those deriving significant value (e.g. large-scale apps in production using LangSmith to improve reliability) pay proportionally, while tinkerers and small teams can often use it free or cheaply, which helps keep the community engaged and growing.
28. CoreWeave: Cloud GPU Provider with Usage-Based Pricing
Background: CoreWeave is a specialized cloud provider offering GPU computing at scale, often at lower prices or greater availability than big clouds. It became especially relevant for generative AI companies in need of lots of GPUs (for model training or inference). They essentially rent out GPUs (like A100s, H100s, etc.) on-demand or via reserved contracts.
Monetization: CoreWeave’s model is straightforward infrastructure-as-a-service:
- They publish hourly rates for various GPU instances. For example, an Nvidia A100 40GB might be, say, $2.50 per hour on-demand. An older GPU like a T4 might be $0.08/hour (just as the search result indicates).
- They offer discounts for reserved or bulk usage (one article mentioned 20-50% cheaper than AWS in general). E.g. a committed contract for a block of GPUs might come down to $1.50/hour for A100 if volume is high.
- They might not charge for other things like storage or networking as primary (though if they provide data storage or other services, those would be billed, but core is GPU hours).
- They also have “burst” pricing or lower priority rates possibly – e.g. lower price for interruptible usage (not sure if CoreWeave does this specifically, but some providers do).
- Essentially usage-based: a customer launches X GPU machines, runs them Y hours, and pays (X * Y * hourly_rate).
- CoreWeave also has professional services or support for enterprise deals, but main revenue is from compute usage.
Why this model: CoreWeave’s clients are often generative AI model training tasks or large inference workloads. The outcome they want is access to high-end GPUs when needed, at a predictable (and ideally lower) cost. Usage-based billing is natural here: you pay for the hardware time you consume, nothing more, which is very transparent.
This is similar to AWS/Azure GPU pricing but CoreWeave competes by:
- Lower absolute prices (as results noted, e.g. $2.39/h vs $3.40/h on Azure for a comparable GPU).
- More flexible terms (willing to do reserved deals at much cheaper rates because they focused on GPU rental business).
- Possibly specialized services (they might have better scheduling for large distributed jobs, etc.).
By undercutting big clouds by say 30-50% on price and focusing on GPU, they appeal to AI startups who find AWS too expensive or capacity-limited. The usage model means those startups can scale up training clusters for a few days (pay a lot during that period), then shut down and pay nothing until next time – very aligned with how AI training is spiky.
They also likely do volume deals: e.g. if a client reserves 100 H100 GPUs for 6 months, they get a much lower hourly rate (because CoreWeave secures revenue and can plan capacity). That aligns with outcomes too – a company needing continuous training capacity might prefer a fixed cost arrangement that guarantees them GPUs. So CoreWeave provides that with hourly rates maybe half of on-demand but you pay regardless of usage (like cloud reserved instances). That’s essentially outcome-based: the client pays a lower price to guarantee outcome (having GPUs available whenever needed for that term).
CoreWeave’s cost structure is basically hardware + electricity + data center costs, so their pricing must cover that plus margin. They probably operate on thinner margins than hyperscalers, focusing on volume. They attracted large clients (like some rumor that even OpenAI or Inflection used them for overflow compute). For those clients, reliability and dedicated supply is critical – so CoreWeave might sign multi-million contracts (like selling X GPU hours at Y rate per hour). It's usage-based at core but with enterprise negotiation.
Because they exclusively do this, they can afford to specialize pricing models. E.g., offering spot pricing: if GPUs are idle, rent them cheaper (like that tweet reference of $0.05 per 4 sec = $0.05 per short render from an X user) might imply some usage stat (though that might be referencing something else). Possibly that was a user measuring effective cost for a Gen-2 video clip on CoreWeave.
Anyway, straight metered pricing means clients pay exactly for compute they use, which ties to how much training or inference they do (the outcome: amount of AI computation done). If a project is big (more computation), it costs more, but presumably that project is more valuable (like training a cutting-edge model). If it's small, the cost is small. This granularity helps startups not waste money when not actively training. It also allows CoreWeave to maximize utilization by adjusting pricing (like encouraging people to use unused capacity via spot rates ensures GPUs rarely sit idle – which is an outcome for CoreWeave: high utilization yields more revenue relative to fixed asset cost).
Additionally, they can differentiate by not charging for some things big clouds do (like hefty data egress fees) making them more attractive cost-wise for heavy data training.
In summary, CoreWeave’s monetization is simply “GPU time as a service”. They succeeded in luring AI companies by linking price directly to the consumption of that critical resource, at rates well below competitors, and offering deals that ensure those who need consistent outcomes (GPU availability) can get it for a predictable price. It’s a classic infrastructure usage model, competing on price-performance. As AI booms, their usage-based revenue scales up massively with demand (they reportedly managed ~250k GPUs, meaning capacity for huge usage; as long as they rent those out, revenue flows in proportionally). It’s a volume business with relatively low margins per unit, but given the skyrocketing need for GPU compute (outcome: model trained or served), the aggregate opportunity is huge.
Segment Summary: GenAI infrastructure companies monetize primarily via B2B services, often usage-based or enterprise contract models. The key patterns:
- Usage-Based Cloud Services: (Hugging Face Inference, Pinecone, CoreWeave) charge by API calls, data stored, or GPU hours. This directly ties revenue to how much underlying resource the customer uses – scaling with the customer’s generative AI workload.
- Open-Source with Enterprise/Cloud SaaS: (Weaviate, LangChain, Hugging Face Hub, etc.) give free community offerings to drive adoption, then convert serious users to paid managed services or support contracts. The monetization kicks in when the outcome needed is enterprise-grade reliability, privacy, or ease-of-use at scale.
- Per-Seat Enterprise SaaS: (Weights & Biases, potentially LangSmith partially) charge based on number of users who benefit from the tool, often layering on usage or storage fees. This fits when the outcome is improved team productivity – they align cost per user who gains that productivity.
- Enterprise Contracts: Many of these infra providers supplement with custom enterprise deals (private deployments, SLAs) because large companies will pay more for tailored outcomes (like guaranteed uptime, security compliance). This often yields high ACVs (Annual Contract Values) aligning with the mission-critical outcome delivered (e.g. W&B ensuring an entire ML org’s experiments are tracked reliably, which is worth six figures to a big enterprise).
Across infrastructure, pricing transparency and flexibility are crucial to win trust of developer and enterprise customers. They often emphasize lower TCO (Total Cost of Ownership) vs DIY or big competitors: e.g. CoreWeave highlighting 20-50% cost savings or Hugging Face implying cost savings by not having to deploy your own inference stack.
Finally, these providers know that their customers (AI startups, dev teams) will succeed or fail often in proportion to how well the infra works and at what cost. So by pricing fairly (usage-based or per-seat for value-add software) and scaling with customers, they ensure customers see the relationship as partnership: if we (the customer) scale our AI usage and succeed, we pay you (the infra provider) more gladly. This alignment fosters long-term relationships and revenue growth along with the GenAI wave.
Applying the Monetizely 5-Step Pricing Framework to GenAI
Pricing a GenAI product to scale requires a holistic approach. We’ll use Monetizely’s 5-Step Pricing Transformation Framework: Goals & Segments, Packaging, Pricing Metric, Rate Setting, and Operationalization, to structure our recommendations for founders:
Step 1: Goals & Segmentation
Identify your highest-value customers and their success outcomes
Start by clarifying who benefits most from your GenAI product and what they’re trying to achieve. Your users may range from hobbyists and students to mid-market companies and global enterprises. Their needs, and willingness to pay, will vary accordingly.
Segment your market along dimensions that reflect meaningful differences in usage and value:
- By company size (e.g., SMBs vs. Enterprises)
- By industry (e.g., legal, healthcare, marketing)
- By usage pattern (e.g., casual creators vs. power users)
For each segment, articulate the primary outcome they seek. Some may want to save time, others to reduce headcount, and others to boost content velocity or decision accuracy. For example:
- Jasper targeted content marketers aiming to produce high volumes of content, fast.
- Character.AI focused on consumer users seeking companionship and entertainment.
Pricing should align with these value drivers. Enterprises may justify high spend if they see quantifiable ROI like revenue uplift or time saved. In contrast, individual users might value emotional connection, novelty, or creativity.
Don’t fall into the trap of one-size-fits-all pricing. If your user base splits across very different needs, it’s better to define a core segment to win and build dedicated plans for adjacent segments. This segmentation lays the groundwork for differentiated packaging and metrics later in the framework.
Step 2: Packaging & Positioning
Create editions that align pricing with perceived value
Once your segments are clear, design packages that bundle the right set of features, usage limits, and service levels for each group. The goal is to present a compelling value proposition at every price point, maximizing willingness to pay.
GenAI products often package across dimensions like:
- Number of seats
- Usage volume (e.g., tokens, credits, minutes)
- Model sophistication (basic vs. advanced models)
- Support tier and enterprise capabilities
Runway ML is a great example: its pricing tiers range from Free (limited features and credits) to Pro (expanded tools and usage) to Unlimited, offering a clear progression for casual creators to upgrade as their needs grow.
Classic Good-Better-Best packaging can still work, but only if thoughtfully executed:
- The “Good” tier should appeal to price-sensitive or trial users
- The “Better” tier should deliver the best value-to-cost ratio, and act as your primary conversion tier
- The “Best” tier should be reserved for those with the highest willingness to pay, offering premium usage, priority support, or exclusive features
To support conversion, each step-up in tier should unlock a meaningful increase in value, more usage, better models, or stronger support. Frame packages around outcomes, not just features: e.g., “Team Plan – Collaborate and generate 3X more content,” or “Enterprise Plan – Maximize ROI with custom AI and white-glove onboarding.” This is especially important in GenAI, where the underlying tech may feel like a black box to many customers.
Step 3: Pricing Metric
Choose a unit of value that scales with customer success
Your pricing metric, what you charge for, is one of the most critical choices you'll make. It must align with the value the customer receives while remaining intuitive and scalable.
Depending on your product, the pricing metric could be based on:
- Input (e.g., API calls, tokens processed)
- Output (e.g., documents, images, conversations)
- Users (e.g., per seat)
- Outcomes (e.g., chargebacks won, leads converted)
The right metric balances fairness (customer pays in proportion to value received) with simplicity. Ask yourself: What does the customer want more of as they succeed? Charge for that.
- OpenAI charges per token, closely mapping cost and value.
- Chargeflow charges per chargeback won, an outcome-aligned metric with direct business value.
For GenAI content tools, metrics like “per word,” “per campaign,” or “per minute of generated video” may be relevant, but beware of overly granular models that feel transactional or inhibit usage. Many GenAI companies now opt for hybrid pricing, combining access-based (per user) and usage-based (per output) components. This allows monetization of heavy users while keeping entry barriers low.
Also consider your own cost structure. If each inference or compute cycle costs you real dollars, make sure your pricing metric reflects that without offloading backend complexity onto the customer. If necessary, abstract usage into credits or minutes to simplify the customer experience.
Finally, validate your metric with real users: Does this feel fair? Does it scale with their success? The best metrics make revenue expansion feel natural, when the customer wins, you win too.
Step 4: Rate Setting
Price based on value delivered, not just costs incurred
With your packaging and metric defined, it's time to set actual price points. Start with market research to understand the price anchors your customers already see, from incumbents, substitutes, or status quo alternatives.
Then work backward from value delivered. If your product saves $10,000 a month in human effort, pricing it at $1,000–$2,000 can be easily justified. Harvey, a GenAI tool for legal workflows, prices at ~$1,000 per user because the value of a good AI suggestion to a lawyer can exceed thousands in billable hours.
Use price psychology to frame your tiers:
- Make the middle tier look like the most popular (and best value)
- Price the entry tier low enough to attract trials or SMBs
- Price the top tier high to signal completeness and premium value
Avoid underpricing your innovation, GenAI tools often deliver significant productivity gains, and customers will pay for those. But also avoid overpricing usage in ways that make customers afraid to engage. If necessary, offer volume discounts or tiered bundles to lower effective unit cost as usage scales. OpenAI reduced per-token prices as usage surged, a smart move to unlock more consumption.
This step will often require iteration. Pilot pricing with a subset of users or run A/B tests in self-serve flows to gather elasticity data. And always connect pricing to outcomes: if the price doesn't feel justified by what the customer is achieving, adjust either the rate or the pricing metric.
Step 5: Operationalization
Execute pricing cleanly, and evolve it as you learn
Pricing is not just a strategy, it’s a system you need to operate and improve continuously.
Start with a clean rollout:
- Update your website, sales decks, and onboarding materials
- Train your sales team with ROI stories, comparison tools, and objection handling scripts
Ensure your backend can support your pricing model. If you charge per usage, your billing and tracking systems must be precise and transparent. Many GenAI companies now show customers usage dashboards, helping build trust and avoid surprises.
Once live, monitor key signals:
- Conversion rates from free → paid or trial → full
- Plan upgrades, usage cap thresholds, and churn drivers
- Feedback from customers about fairness or friction
Stay responsive. If customers are frequently hitting usage caps and disengaging, consider introducing “bridge” tiers. If enterprise buyers want one high-end feature but not the full package, consider unbundling it as an add-on.
Expect to evolve pricing as your product matures. You may need to:
- Introduce a new entry tier to drive volume
- Reprice a high-value feature and grandfather existing customers
- Shift from flat-rate to usage-based pricing on some modules
Just make sure any change is anchored in improved value delivery, and communicated transparently. Customers are willing to accept change when it’s clear they’re getting more in return.
Lastly, embed pricing into your product’s experience:
- Show usage counters and upgrade prompts in-app
- Make it self-serve to upgrade or change plans
- Reinforce value continuously through UI and messaging
In Closing
The monetization of generative AI from 2023 to 2025 has been defined by flexibility, scalability, and outcome-orientation. Companies are charging in ways that feel intuitive relative to the value delivered, whether that’s per document summarized, per user assisted, or per model deployed.
As the market matures, pricing is becoming more precise. We're seeing:
- Usage-based layers added to seat-based models. For instance, Weights & Biases could begin charging by experiment storage, or LangSmith by the number of model calls tested beyond a threshold.
- Bundled pricing for heavy usage scenarios. Anthropic’s “unlimited throughput” subscriptions and OpenAI’s flat-fee enterprise plans offer predictable costs while ensuring a certain outcome level.
These shifts mirror established SaaS pricing frameworks, now applied to GenAI:
- Tiered Value Metric: Pricing based on units of value, seats, tokens, credits, words, images, minutes, or vectors, tiered to scale with customer need.
- Land and Expand: Low-friction entry followed by expansion as usage grows (e.g., Jasper moving from single-seat to team plans, or Pinecone scaling from one index to many).
- Outcome-Based Messaging: Even when not purely outcome-priced, marketing highlights ROI: “100 images for the cost of a coffee” or “save X hours, achieve Yx ROI.”
- Continuous Iteration: Pricing adjusts with product evolution and market shifts. Stability AI’s price cuts forced others to revisit image pricing; OpenAI’s reductions pressured API rivals. In AI, pricing must remain agile as costs fall and open-source alternatives emerge.
Across these 28 GenAI firms, one principle stands out: charge for the magic, but make sure the customer feels they’re getting a magical deal. That balance drives adoption, funds innovation, and builds lasting trust as generative AI becomes more embedded in products and workflows.
Each category, SaaS, APIs, consumer tools, infrastructure, interprets this principle differently, but all aim to align pricing with outcomes that matter to their customers
Follow Monetizely's 5-step pricing framework, and your pricing won’t just support your business model, it will amplify it. To learn more about our pricing strategies, feel free to book a free pricing assessment with our pricing experts.